Kindly fill up the following to try out our sandbox experience. We will get back to you at the earliest.
Data Trust for the AI era
As the reliance on data increases due to the demand for GenAI, it is imperative that we rethink the new framework that can support our data management strategies.
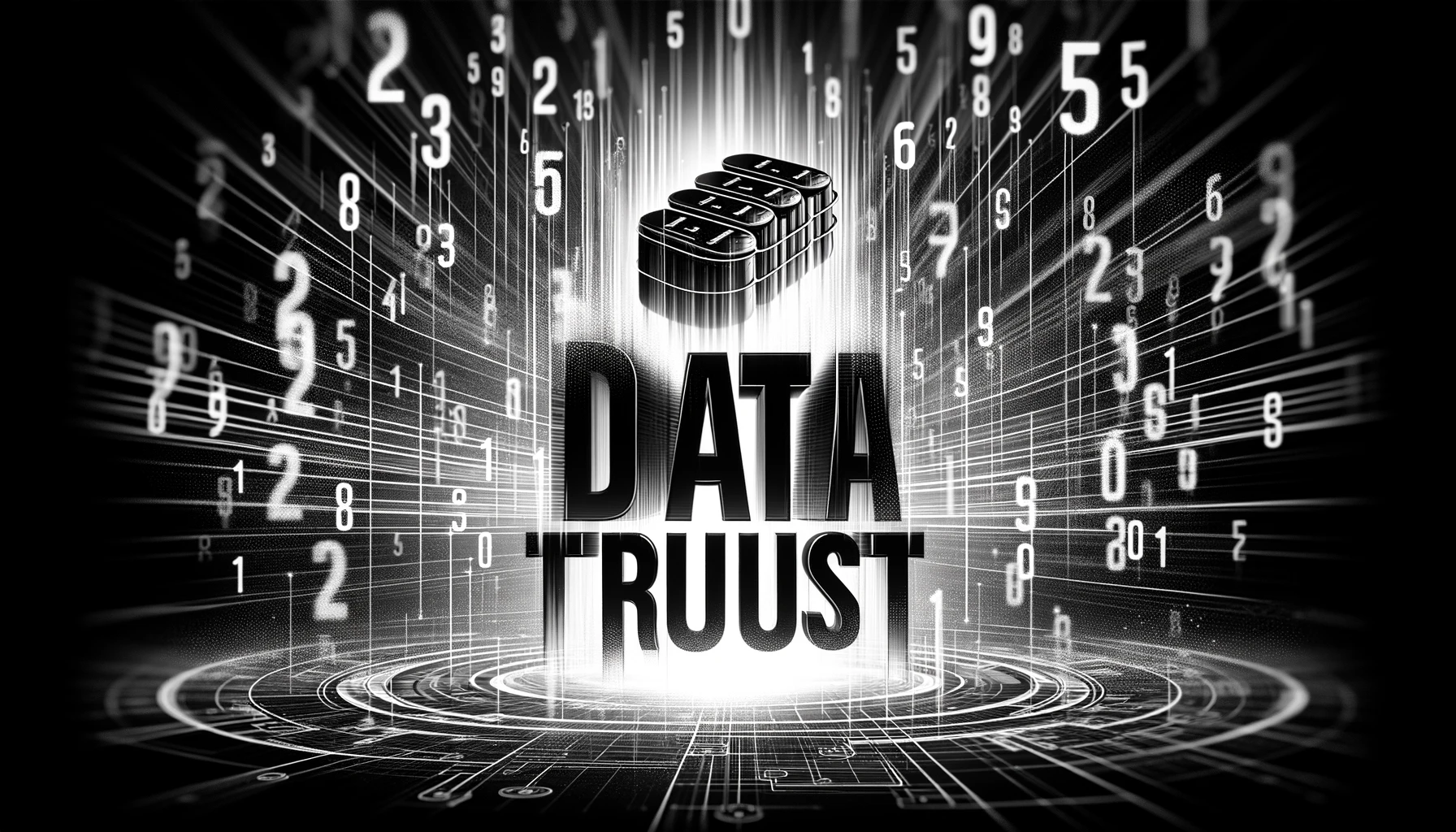
As we enter the era of AI, data trust is more important than ever. Data governance, catalog, and quality frameworks existed for a long time, but let’s face it: AI is changing the game and is more demanding. it’s high time we rethink on the framework which can help the data team drive reliability across the value chain.
Let’s be real: it’s easy to get swept up in the hype of using popular tools like Databricks, Snowflake, or Apache Iceberg. But at the end of the day, what truly matters is building trust in our data/AI products. Businesses often face challenges with visibility, traceability, and confidence in their data, and it’s up to data teams (you know, the engineers, scientists, AI gurus, and analysts) to deliver reliable and secure solutions.
As the collaboration between data and business teams has grown wider, so has the potential for conflict. Meetings can become a battleground, with discussions focusing on assigning blame for issues like low-quality dashboards or inconsistencies in output. This dynamic undermines teamwork and stifles progress.
Conventional Method
Over the last decade, numerous technologies have been introduced to make perfect spaghetti. Complications arise when these tools don’t integrate seamlessly, resulting in data-tool debt. Its not about just integration but also seamless flow of information and burden of onboarding and integrating data sources again and again.
Earlier methods were quite manual and resource-intensive; there are times where we have witnessed data quality being checked once a week / month.
As the complexity and volume of data continue to grow exponentially, traditional data management approaches are struggling to keep up. Conventional technologies often fall short when it comes to handling the scale and intricacy of modern data ecosystems. Data quality, observability, cataloging, and governance are all crucial aspects of effective data management, yet these components are frequently siloed and disjointed in legacy systems. This fragmentation can lead to data inconsistencies, governance challenges, and increased risk.
Moreover, traditional technologies may not be equipped to handle the real-time nature of today’s data-driven world. Today, companies are leveraging streaming technologies like Kafka, AWS MSK, and Google Pub/Sub; this is extremely challenging, or even these technologies are not supported by old-tech/ platforms.
What's the new framework around data trust?
The Data Trust framework consists of several interconnected components that work together to create a solid foundation for data management:
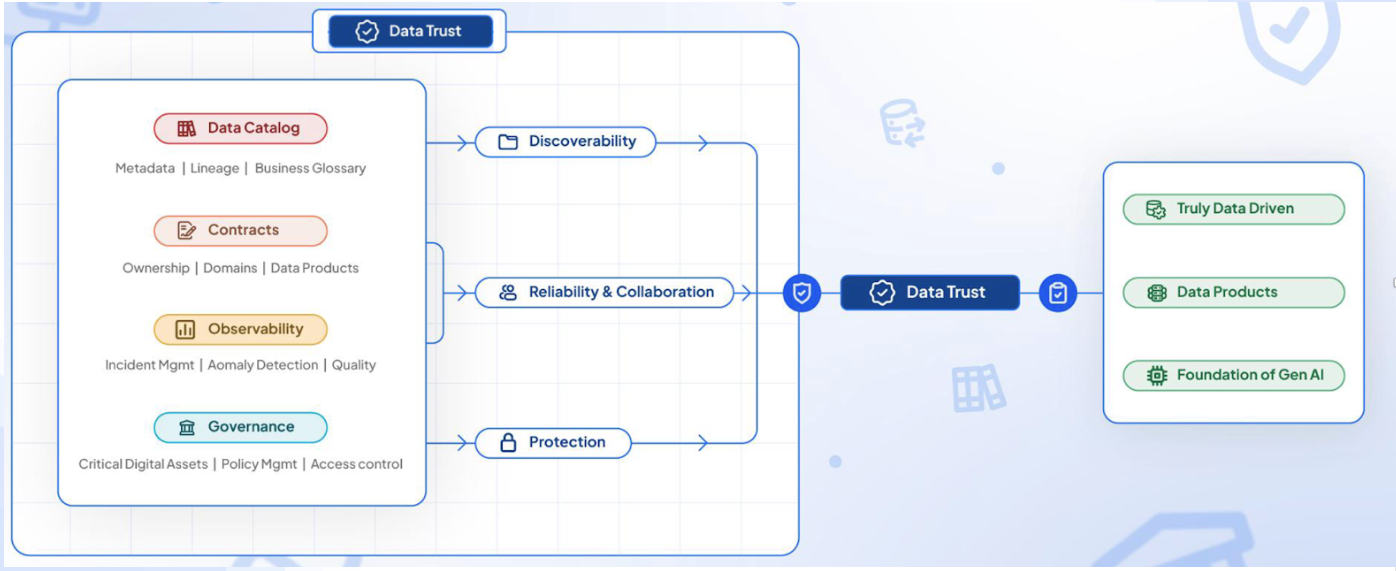
- Data Discovery and Metadata Management: The first step in implementing a Data Trust framework is to understand the breadth of your data sources and the information available. Data discovery and metadata management provide valuable insights into your data landscape, enabling organizations to make informed decisions about data usage and prioritization.
- Data Domain Classification and Prioritization: To ensure that your organization focuses on its most critical data elements, classify data into relevant domains and mark them based on usage and importance. This helps in building effective data products and ensures that your data efforts align with your business objectives.
- Data Observability for Reliability and Performance Monitoring: Implementing data observability is essential for monitoring data pipelines and ensuring data reliability. By detecting and mitigating data incidents quickly, organizations can significantly improve data quality and maintain the trust of their stakeholders.
- Enhanced Collaboration and Data Contracts: Establishing data contracts is crucial for improving collaboration between data teams and business stakeholders. These contracts outline expectations and responsibilities, fostering trust and accountability across the organization.
- Data Governance Strategies: Finally, protect critical data elements by applying appropriate governance strategies. This ensures that sensitive information remains secure and that only authorized personnel can access it.
The next question that can tinker with the brain is: I will build it on my own.
The Buy vs. Build Dilemma
When implementing a Data Trust framework, organizations must decide whether to buy an existing solution or build one in-house. While each approach has its merits, several factors should be considered before making a decision.Building a Data Trust FrameworkBuilding a Data Trust framework from scratch offers greater control and customization but comes with several challenges:
- High development costs: Developing a comprehensive Data Trust framework requires significant investments in resources, technology, and expertise.
- Time-consuming: Building a framework in-house can take months or even years, diverting focus from core business activities.
- Maintenance and updates: In-house frameworks require ongoing maintenance and updates to stay current with evolving data management trends and regulatory requirements.
Buying a Data Trust Framework
Opting for a pre-built Data Trust platform can be more cost-effective and efficient.
- Faster implementation: Pre-built solutions can be deployed quickly, enabling organizations to realize the benefits of a Data Trust framework in a shorter timeframe.
- Expertise and support: Vendors often provide expert guidance and ongoing support to ensure the successful implementation and maintenance of the framework.
- Lower costs: Buying a pre-built framework can be more cost-effective in the long run, as organizations avoid the high development costs associated with in-house solutions.
Let me buy individual solution
As organizations strive to improve their Data Trust strategies, many find themselves grappling with the challenges of siloed data platforms. While implementing standalone solutions, such as data catalogs and data observability tools, may initially seem like a viable approach, it often leads to integration issues, increased vendor dependency, and disconnected systems. Let’s explore these disadvantages in detail:Disadvantages of Siloed Data Platforms
- Seamless Integration Challenges: When data platforms operate in isolation, integrating them into a cohesive system can be a daunting task. The lack of unified architecture and standardization can lead to compatibility issues, data inconsistencies, and additional development work to bridge the gaps between systems.
- Dependency on Multiple Vendors: Relying on multiple vendors for different aspects of your Data Trust strategy can result in increased costs, complex contract negotiations, and potential vendor lock-in. Additionally, coordinating support and updates across various vendors can be time-consuming and inefficient.
- Disconnected Platforms: Perhaps the most significant drawback of siloed data platforms is the lack of communication between systems. When data catalogs, data observability tools, and other platforms don’t “talk” to each other, it becomes challenging to maintain data consistency, identify potential issues, and ensure the reliability of data assets.
The Unified Solution: Breaking Down Silos
To overcome the limitations of siloed data platforms, organizations can adopt a unified solution that brings together data cataloging, data observability, and other essential components of Data Trust management. By consolidating these functions into a single platform, businesses can enjoy the following benefits:
- Seamless Integration: A unified solution is built on a single architecture, ensuring that all components work together harmoniously. This eliminates integration challenges and promotes data consistency across the organization.
- Reduced Vendor Dependency: With a unified platform, organizations can minimize their reliance on multiple vendors, reducing costs and streamlining vendor management processes. This also ensures a more consistent and cohesive support experience.
- Enhanced Communication: A unified solution enables data catalogs, observability tools, and other components to communicate effectively, providing a holistic view of the data landscape. This leads to more informed decision-making, proactive issue identification, and improved data reliability.
Final Thoughts:As we conclude our exploration of the data Trust landscape, it’s evident that a comprehensive and unified approach to data management is vital for success in today’s data-driven world. By addressing the key components of Data Trust, including data quality, observability, cataloging, and contracts, organizations can foster collaboration, ensure data reliability, and drive innovation.Embracing a modern, scalable solution is crucial to overcoming the limitations of conventional technologies and effectively navigating the complexities of AI and digital transformation. With a solid Data Trust framework in place, businesses can capitalize on the immense opportunities that lie ahead, bolstering their competitive edge and paving the way for long-term growth and success.
FAQs:
- What is data trust, and why is it important? Data Trust refers to the reliability, accuracy, and trustworthiness of an organization’s data. It’s essential for making informed decisions, fostering collaboration, and ensuring the success of AI and digital transformation initiatives.
- What are the key components of a Data Trust framework? A comprehensive Data Trust framework should address data quality, observability, cataloging, and contracts, among other elements. These components work together to ensure data reliability and effective data management.
- Why are conventional technologies insufficient for modern data management? Traditional data management approaches often struggle to handle the scale and complexity of today’s data ecosystems. They may lack the agility, adaptability, and unified architecture required to meet the demands of real-time data monitoring and AI-driven innovation.
- How can organizations overcome the challenges of Data Trust? By adopting a unified, scalable solution that effectively integrates data quality, observability, cataloging, and contracts, organizations can overcome the limitations of conventional technologies and build a solid foundation for Data Trust. This enables them to harness the power of AI and drive growth in the digital era.