Kindly fill up the following to try out our sandbox experience. We will get back to you at the earliest.
What is Data Observability? A Comprehensive Guide
Discover how data observability enhances data reliability, quality, and informed decision-making for organizations

What is Data Observability?
Hey there, data engineers and data enthusiasts! You might have heard the term "data observability" buzzing around lately, and if you're curious to know more, you're in the right place. This article aims to break down what data observability is all about and share some techniques that'll help you and your data team harness its full potential.
But first, let's get a grasp on what data observability actually means.
Data Observability Concept Explained
Data observability is a crucial concept for organizations seeking to ensure the reliability and accuracy of their data. It involves having a comprehensive understanding of the health and performance of the data within their systems. By using automated monitoring, root cause analysis, data lineage, and data health insights, organizations can proactively detect, resolve, and prevent data anomalies.
It’s the framework to monitor the health of your data on a real-time basis and ensure data reliability which can then be input to Data or GenAI products.
The emerging field of data observability is gaining significant traction in the landscape of data management and analytics. At its essence, data observability refers to the ability to fully understand and effectively manage the health of an organization's data ecosystem. This concept extends beyond traditional monitoring, offering a more holistic view of the data's integrity, availability, and reliability. By implementing data observability, businesses can proactively identify and address data issues, ensuring that their data remains accurate, consistent, and trustworthy. This is especially crucial in today's data-driven world, where the quality of data directly impacts decision-making and operational efficiency
The five pillars of data observability - freshness, quality, volume, schema, and lineage - provide valuable insights into the quality and reliability of the data. Freshness measures the timeliness of data updates, while quality assesses the accuracy and consistency of the data. Volume evaluates the completeness of data tables, and schema examines any changes in data organization. Lineage provides essential information on the upstream and downstream impact of data issues.
Implementing data observability tools is key to improving data pipelines, increasing team productivity, and enhancing overall data management practices. These tools enable organizations to have confidence in their decision-making processes and deliver high-quality data for analysis. Ultimately, data observability ensures improved customer satisfaction by maintaining good data quality and integrity.
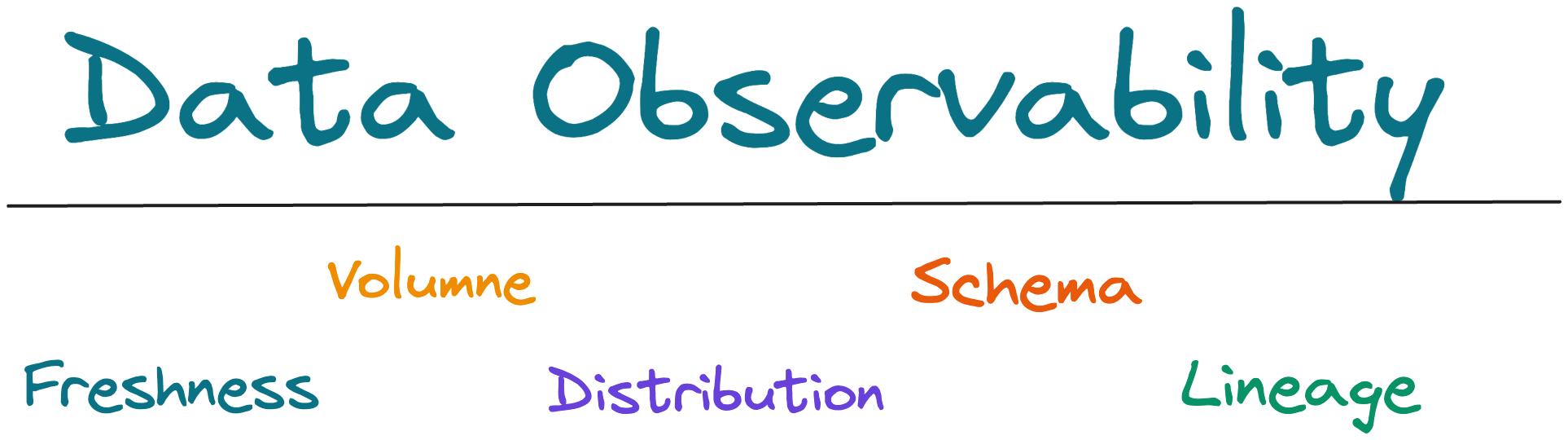
Key Takeaways:
- Data observability involves understanding the health and performance of data within an organization's systems.
- The five pillars of data observability - freshness, quality, volume, schema, and lineage - provide insights into data quality and reliability.
- Data observability tools improve data pipelines, team productivity, and customer satisfaction.
- Implementing data observability requires automated monitoring, root cause analysis, and data health insights.
- Data observability helps organizations make informed decisions based on high-quality data.
5 Pillars of Data Observability
Data observability relies on five key pillars to provide organizations with valuable insights into the quality and reliability of their data. These pillars, namely freshness, quality, volume, schema, and lineage, play a crucial role in ensuring comprehensive data observability and supporting data-driven decision-making.
Freshness
This pillar focuses on the timeliness of data updates. By monitoring the freshness of data, organizations can ensure that their insights and analyses are based on the most up-to-date information available.
Quality
The quality pillar assesses the accuracy and consistency of the data itself. Organizations can rely on data observability to detect and resolve issues related to data quality, ensuring that they have access to reliable and trustworthy data for decision-making.
Volume
Measuring the completeness of data tables, the volume pillar allows organizations to determine if they have sufficient data for their analyses. By ensuring that data tables are adequately populated, organizations can avoid making decisions based on incomplete or insufficient information.
Schema
The schema pillar examines changes in data organization. Organizations can leverage data observability to monitor and track schema modifications, ensuring that data remains structured and consistent across different systems and sources.
Lineage
Providing information on the upstream and downstream impact of data issues, the lineage pillar offers valuable insights into the origin and flow of data. With data observability, organizations can track the lineage of data, helping them identify and address potential data dependencies or issues that could impact data reliability.
By considering these five pillars of data observability, organizations can achieve a comprehensive understanding of their data, ensuring its quality, reliability, and integrity. This, in turn, empowers organizations to make informed decisions based on accurate and trustworthy data.
The Importance of Data Observability
Data observability is crucial for organizations as it ensures the reliability and accuracy of data. By monitoring the health of data, organizations can have confidence in their decision-making processes and deliver high-quality data for analysis. Data observability helps identify and prevent data downtime, which can lead to wasted time and resources.
In today's data-driven world, where organizations rely on complex data systems that support multiple data sources and consumers, data observability plays a vital role. It enables organizations to maintain good data quality, enhance data integrity, and improve data operations.
When data systems become more complex, it becomes increasingly challenging to ensure the reliability and accuracy of data. Data observability provides organizations with the necessary tools and insights to proactively identify and resolve data issues, minimizing the impact on data quality. By continuously monitoring data health, organizations can detect anomalies, streamline data operations, and ensure that their data is consistently reliable.
Establishing a strong data observability architecture is critical to reaping the benefits of data observability. This architecture is a framework that incorporates numerous technologies and procedures to monitor, analyze, and manage a large amount of data within an organization. Data pipelines, databases, and storage systems are integrated, along with advanced analytics, to deliver real-time insights on data health. The design seeks to generate a continuous flow of information across multiple layers of the data stack, allowing for quick identification and resolution of data problems. By implementing a well-designed data observability architecture, enterprises may ensure data quality and integrity throughout their data landscapes.
Benefits of Data Observability:
- Enhanced decision-making processes
- Improved data quality and reliability
- Optimized data operations
- Enhanced data integrity
- Increased customer satisfaction
Data observability empowers organizations to make data-driven decisions with confidence and unlock the full potential of their data. It allows businesses to identify data issues, prevent data downtime, and ensure that their data operations are running smoothly. With data observability, organizations can maintain high data quality, improve data integrity, and enhance overall data operations.
Data observability is not just a nice-to-have feature; it is a critical component of modern data management. As organizations continue to leverage data to gain a competitive edge, implementing data observability becomes essential to ensure the reliability and accuracy of the underlying data.
Key Features of Data Observability Tools
Data observability tools offer a range of features that are essential for effective data management. These tools seamlessly connect to existing data stacks without the need for any modifications to data pipelines or code. By providing robust monitoring capabilities for data at rest, they ensure scalability, performance, and compliance with security and regulatory requirements.
One key feature of data observability tools is their utilization of machine learning models. These models automatically learn the data environment and detect anomalies, minimizing false positives and allowing organizations to proactively identify and resolve data issues. Additionally, data observability tools provide rich context that facilitates rapid troubleshooting and effective communication with stakeholders, ensuring quick and informed decision-making.
Data observability metrics are at the heart of the approach. These metrics are critical indications for enterprises to analyze and evaluate the performance, quality, and dependability of their data. Common measurements include data freshness, volume, mistake rates, and pipeline delay. These quantitative metrics of observability are obtained from a variety of data sources, including operational databases, data warehouses, and real-time data streams. Analyzing these indicators may provide companies with useful insights into the health of their data systems, allowing them to make educated choices and take prompt action to avoid risks related with data quality and compliance.
With data observability tools, organizations can maintain data reliability and prevent issues before they impact critical operations. By leveraging the power of these tools, organizations can enhance their data monitoring practices, ensuring the accuracy, consistency, and integrity of their data.
Data observability tools offer seamless integration, advanced monitoring capabilities, and the ability to automatically detect anomalies. This enables organizations to maintain data reliability and prevent issues before they impact critical operations.
Data Observability vs. Data Quality
Data observability and data quality are two essential aspects of effective data management. While data quality focuses on the accuracy, consistency, and validity of data, data observability provides visibility into the overall health and state of data in a system. Both concepts work together to ensure data reliability, which is crucial for making informed decisions and driving successful outcomes.
Data quality ensures that the data is trustworthy, free from errors, and aligned with predefined standards. It focuses on validating data against specified rules, cleaning and transforming data when necessary, and ensuring the data is consistent and complete. Data quality processes aim to maintain and improve the accuracy, integrity, and relevance of the data, playing a critical role in decision-making processes.
Data observability, on the other hand, provides a holistic view of the data environment, enabling organizations to monitor, detect, and resolve data-related issues. It embraces a proactive approach to data management by continuously monitoring the health and performance of data pipelines, identifying anomalies, and ensuring data aligns with predefined expectations. Data observability helps organizations gain insights into the overall quality, reliability, and usability of data, allowing them to address any potential issues before they impact data quality.
"Data observability and data quality work hand in hand to ensure accurate, reliable, and trustworthy data"
To better understand the difference between data observability and data quality, let's compare them using a table:
By considering the unique strengths of data observability and data quality, organizations can establish a comprehensive data management strategy that ensures the reliability, quality, and usability of their data assets.
Key Takeaways:
- Data observability focuses on the overall health and state of data, while data quality ensures the accuracy and consistency of individual data elements.
- Data observability enables organizations to proactively monitor data pipelines and detect anomalies, while data quality validates data against predefined standards and rules.
- Data observability provides visibility into the overall data environment, while data quality focuses on the individual data elements.
- Both data observability and data quality are essential for ensuring data reliability, integrity, and usability.
Implementing Data Observability
Implementing data observability is a crucial step for organizations seeking to ensure data quality, streamline data pipelines, and establish robust data governance practices. By integrating data observability tools into their existing data infrastructure, organizations can proactively monitor and manage the health and reliability of their data, improving overall data operations.
To implement data observability, organizations need to:
Integrate Data Observability Tools:
This entails connecting data observability tools to data pipelines, allowing for real-time monitoring and analysis of data quality, freshness, and performance.
Establish Data Governance Practices:
Developing clear data governance policies and procedures is essential for maintaining data integrity and ensuring compliance with regulations. Organizations should define metrics, thresholds, and data stewardship roles to effectively monitor and enforce data governance practices.
Define Metrics for Monitoring:
By defining relevant metrics and thresholds, organizations can monitor the health and performance of their data pipelines. This includes measuring data freshness, quality, and volume, enabling early detection of anomalies and potential issues impacting data reliability.
Understand Data Sources and Dependencies:
It is crucial for organizations to have a clear understanding of their data sources, data lineage, and dependencies. This knowledge allows for effective troubleshooting, root cause analysis, and resolution of data issues.
Implementing data observability empowers organizations to proactively identify and address data abnormalities, ensuring the reliability and accuracy of their data. By monitoring data pipelines, organizations can detect and resolve issues before they impact critical operations. This, in turn, enhances decision-making, improves customer satisfaction, and optimizes overall data management.
Challenges of Data Observability
Data observability brings valuable insights into the health and reliability of data within an organization's systems. However, implementing data observability can pose challenges, especially when dealing with data silos and varying data standards. Let's explore the key challenges in more detail:
Data Silos
Data silos occur when data is isolated within specific departments or systems, limiting data accessibility and visibility. Integrating all data sources and systems into a unified observability platform can be a complex undertaking. Organizations must break down these silos and establish seamless data integration to achieve comprehensive data observability.
Data Standardization
Data standardization is crucial for effective data observability. However, organizations often face challenges in standardizing telemetry data and logging guidelines across different systems. Inconsistent data formats and structures hinder the ability to correlate information accurately. Standardizing data requires careful planning and collaboration among data teams to ensure consistent and reliable data observability.
Storage Costs and Scalability
Data observability involves monitoring and analyzing large volumes of data. Depending on data storage and retention policies, storage costs and scalability can become concerns. Organizations need to assess their storage infrastructure and optimize it to handle the increasing volume of observability data effectively. Scalable and cost-effective data storage solutions are essential for achieving comprehensive data observability.
Collaboration and Approach
Overcoming the challenges of data observability requires a well-planned approach and collaboration among data teams. Organizations need to define clear goals, establish cross-functional teams, and leverage the expertise of data engineers, data scientists, and other stakeholders. Effective collaboration ensures comprehensive data observability and enables organizations to proactively manage their data with confidence.
"Implementing data observability brings numerous benefits, but organizations must be prepared to address the challenges that come along with it. By breaking down data silos, standardizing data, optimizing storage, and fostering collaboration, organizations can overcome these challenges and harness the power of comprehensive data observability."
Next, we will explore the future of data observability and the role it will play in organizations' data strategies.
The Future of Data Observability
Data observability is poised to play a crucial role in the future of data management and operations. With the exponential growth of data generation and processing by organizations, the need for comprehensive data observability is only going to increase. However, the future of data observability is not just about the quantity of data but also about the quality and efficiency of its management.
Advancements in automation, machine learning, and AI will further enhance the capabilities of data observability tools. Organizations will have access to real-time analytics that provide immediate insights into data health, performance, and anomalies. This predictive approach will enable them to identify and resolve data issues proactively, preventing potential disruptions to data operations.
The future of data observability also involves empowering users with self-service data access. This means that data consumers will have greater visibility and control over the data they need, reducing dependencies on data operations teams. Self-service access enables faster analysis, decision-making, and innovation, contributing to improved overall data management efficiency.
Data observability will continue to evolve as organizations strive for a holistic view of their data ecosystem. It will go beyond monitoring and detection, encompassing data governance practices that ensure data quality, compliance, and security. The integration of data observability with data management platforms will provide a seamless experience for organizations, enabling them to leverage the true potential of their data assets.
The role of data observability in the future includes:
- Real-time analytics for immediate insights
- Proactive identification and resolution of data issues
- Self-service data access for faster analysis and decision-making
- Data governance integration for comprehensive data management
Wrapping Up
Data observability is a fundamental concept in modern data management that organizations must implement to ensure the quality and reliability of their data. By integrating data observability tools, establishing data governance practices, and monitoring data health and performance, organizations can proactively manage their data and make informed decisions. Despite the challenges that may arise, data observability brings numerous benefits, including improved data quality, enhanced data operations, and better data management.
As the volume and complexity of data continue to increase, implementing data observability becomes increasingly important. Organizations need to have a comprehensive understanding of the health and performance of their data to maintain its quality and reliability. Data observability allows them to identify and resolve issues, prevent data downtime, and optimize data operations, ultimately leading to improved business outcomes and customer satisfaction.
In conclusion, organizations should prioritize the implementation of data observability to ensure the accuracy, consistency, and validity of their data. By leveraging data observability tools and practices, organizations can achieve data-driven decision-making and effectively manage their data in the face of evolving technologies and data landscapes. Implementing data observability is a critical step in harnessing the full potential of data and staying ahead in today's data-driven world.
FAQ's
What is Data Observability?
Data Observability is a set of practices and technologies that provide real-time monitoring and automated insight into the health and performance of data systems, ensuring data quality, reliability, and accessibility.
What are the five pillars of Data Observability?
The five pillars of Data Observability include Freshness, Distribution, Volume, Schema, and Lineage, which together provide a comprehensive view of data health and performance.
How does Data Observability benefit an organization?
Data Observability enables organizations to proactively identify and resolve data issues, maintain high data quality, enhance decision-making, and build trust in data among stakeholders.
What challenges are addressed by implementing Data Observability?
Data Observability addresses challenges like data downtime, poor data quality, inefficient data operations, and lack of visibility into data systems and workflows.
How does Data Observability differ from Data Monitoring?
While Data Monitoring focuses on tracking system performance and operational metrics, Data Observability provides a deeper, holistic insight into data health, quality, and usage patterns.
What is the role of AI and Machine Learning in Data Observability?
AI and Machine Learning play a crucial role in Data Observability by enabling predictive analytics, anomaly detection, and automated insights, which help in proactive data management.
How does Data Observability support Data Governance?
Data Observability enhances Data Governance by providing transparency, ensuring data compliance, and maintaining data quality and lineage, which are essential for effective governance.
What are the key metrics to track in Data Observability?
Key metrics include data freshness, data volume trends, error rates, schema changes, and data lineage accuracy, which collectively indicate the health of data systems.
What are the common tools and technologies used in Data Observability?
Common tools include data cataloging software, automated monitoring systems, anomaly detection tools, and platforms that integrate AI for advanced analytics and insights.
What is the future outlook for Data Observability?
The future of Data Observability lies in further integration with AI and ML for predictive insights, expansion in cloud and distributed environments, and an increased focus on user-friendly interfaces for broader adoption.
Additional Resources:
- Best Practices in Data Engineering(https://www.forbes.com/sites/forbesbusinesscouncil/2023/01/13/implementing-data-engineering-best-practices/)
- The Rise of Data Observability: Why It Matters and How to Implement It (https://towardsdatascience.com/the-rise-of-data-observability-why-it-matters-and-how-to-implement-it-df3ba3d5e5ee)
- Data Observability: What It Is and Why You Need It (https://medium.com/@DataKitchen/data-observability-what-it-is-and-why-you-need-it-6c03d8839b33)
By staying informed about the latest developments in data observability and continuously evaluating your organization's data reliability, you can maintain a competitive edge and ensure the success of your data-driven initiatives.