Kindly fill up the following to try out our sandbox experience. We will get back to you at the earliest.
Building a Modern Data Stack: Discussion with Piyush Palkar, CDO of Carsome
In our next blog, we interview Piyush from Carsome, exploring his insights on constructing a Modern Data Stack. Expect discussions on cutting-edge data tools, strategies for data integration, and practical steps towards efficient data infrastructure. Don't miss this enlightening conversation that promises to upgrade your data proficiency.
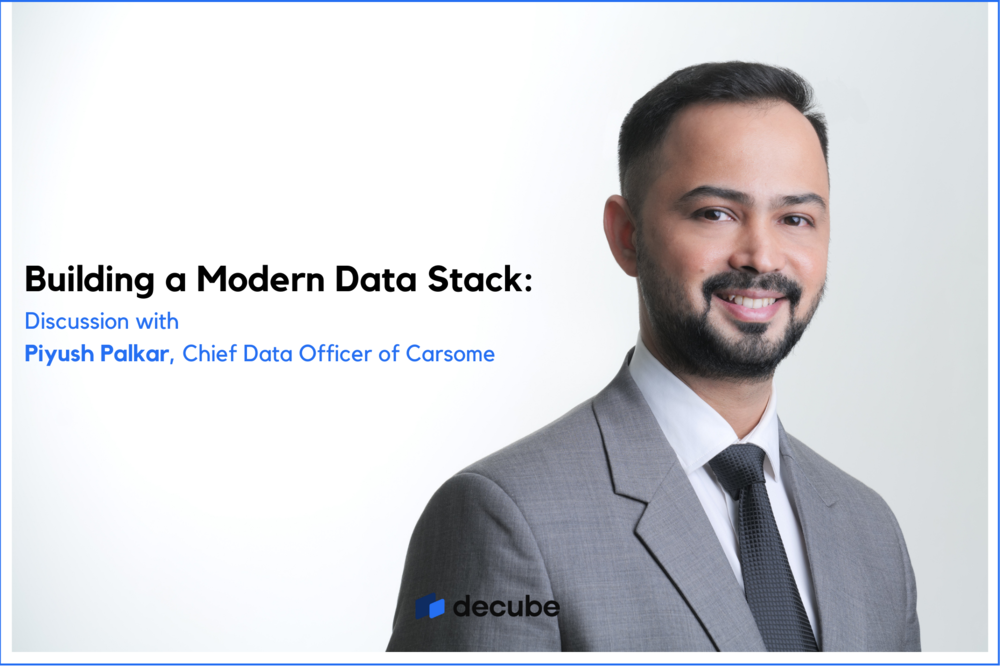
Hello there, my fellow data enthusiasts! We are back at it again with a hot topic that has been keeping me and likely many of you awake at night: “How to build a modern data stack”. To help illuminate this multifaceted concept, I’ve sat down with the brilliant Piyush Palkar, Chief Data Officer at Carsome, a powerhouse in the realm of integrated car e-commerce in Southeast Asia.
What makes Carsome's approach noteworthy is their robust, scalable data solution that is driving an array of both internal and external products. With Piyush at the helm of their data operations, they are pushing the boundaries and setting a fantastic example in the industry.
The Crux: Data Warehousing
Data warehousing is essentially the backbone of your modern data stack. “Think of a data warehouse as the engine of a car, the powerhouse that drives all data activities. It needs to be robust, efficient, and scalable,” Piyush pointed out. This powerful analogy sheds light on the central role that data warehousing plays in handling the vast, complex data operations of an organization like Carsome.
Considering the sheer volume of data generated in the digital age, Carsome decided to lean towards a cloud-native data warehouse, a choice borne out of the need for scalability and performance. When you're dealing with massive datasets that are continually growing, the scalability offered by cloud data warehouses like Snowflake, Databricks, BigQuery, or Redshift can be a lifesaver.
But remember, there isn't a one-size-fits-all solution here. The choice of a data warehouse depends on several factors such as data volume, velocity, variety, and the unique business needs of your organization. Each data warehouse comes with its strengths and weaknesses. Your decision should be based on a clear evaluation of your requirements and the features and capabilities of the potential platforms.
Fueling the Engine: ETL Processes
With your data warehouse set up, you'll need to fill it up with data. And that's where ETL (Extract, Transform, Load) processes come in. Piyush stressed the importance of well-structured ETL pipelines to ensure that data is correctly extracted from various sources, transformed into a suitable format, and loaded into the warehouse without errors or inconsistencies.
Carsome uses a combination of home-grown ETL pipelines and third-party tools. "The choice of tool often comes down to the specific requirements of the job at hand," Piyush explains. Reliable ETL services like Stitch, Fivetran, or Talend are staples in their toolset. However, they aren't shy about developing custom solutions for specific data transformations that may not be addressed by these platforms.
What’s crucial is the scalability of your ETL processes. As your data grows, your ETL processes should be capable of handling this growth without compromising data accuracy or consistency. This scalability is particularly vital in today’s data-driven business environment where data volumes are exploding.
The Maestro of Data: Data Orchestration
In the complex symphony that is a modern data stack, you’ll need a capable conductor to keep everything harmonious. That’s where data orchestration comes into play. “Data orchestration can be seen as the conductor of an orchestra, coordinating various sections (or in our case, data pipelines) to produce a harmonious output,” Piyush analogized.
At Carsome, Apache Airflow serves as this conductor. The decision to use Airflow was primarily due to its flexibility and its excellent handling of complex dependencies between tasks. However, there are other viable options like Dagster or Prefect, which might serve your needs better.
The key takeaway here is to be mindful of your team's skillset and the complexity of your data pipelines. The right orchestration tool can dramatically simplify your data management and improve productivity and efficiency.
Making Sense of the Data: Business Intelligence (BI)
With your data warehouse filled with processed data, it's time to gain insights from it. That’s where your Business Intelligence (BI) tools step in. They're akin to your car's dashboard, providing a snapshot of how your engine (data warehouse) is performing.
In Carsome’s BI ecosystem, they have embraced a mixed approach. "We use Tableau for its advanced data visualization capabilities" shared Piyush. It's important to remember that the choice of a BI tool is largely dependent on factors such as cost, user-friendliness, integration capabilities, and the specific needs of your organization. Other popular choices include Looker and Qlik.
But no matter what tool you use, your aim should be to transform your raw data into meaningful insights that can drive decision-making and strategy development.
The Watchful Guardian: Data Observability
One of the more interesting points that Piyush highlighted during our discussion was the role of data observability. As data volumes increase, the challenges in maintaining data quality and integrity grow correspondingly. "Data observability helps us identify and address issues proactively. It's like a watchdog that keeps a constant eye on our data," Piyush explained.
At Carsome, they use Decube for their data observability needs. Its automated anomaly detection, data lineage tracing, and comprehensive dashboards offer a robust and reliable way of ensuring the health and integrity of their data stack. Other viable platforms in the market include Monte Carlo and Soda. This level of observability may not be an immediate necessity for smaller organizations, but as you scale up, investing in a data observability tool can significantly enhance the quality and reliability of your data.
What's next:
Piyush's extensive expertise extends far beyond building a modern data stack. His wealth of experience in MLOps, data science, and vector database is particularly noteworthy and will be at the forefront of our follow-up interview. This in-depth exploration will delve into his hands-on experience and innovative strategies, offering readers a deep dive into these critical aspects of data handling and operation. Prepare for a comprehensive expose of Piyush's multifaceted skillset in the next installment of our series.
Final Thoughts
Creating a modern data stack is a strategic process that requires careful thought and planning. It's not about finding the "perfect" tool or platform, but rather, identifying what works best for your unique needs and context.
As Piyush wisely advised, "Stay flexible, experiment, and adapt. The data world is evolving rapidly, so our approach to building and managing our data stack needs to evolve as well."
Building your data stack is like a road trip. You'll need a robust engine (data warehouse), a reliable fueling process (ETL), a skilled guide (data orchestration), an informative dashboard (BI), and a vigilant health monitoring system (data observability). It's a journey with challenges and uncertainties, but the destination - a data-driven organization that can leverage insights to drive growth and innovation - is worth the effort.
In the words of Piyush, "Gear up and enjoy the ride!"