Kindly fill up the following to try out our sandbox experience. We will get back to you at the earliest.
Gen AI and the future of Data engineering
Data engineering, the practice of collecting, transforming, and organizing data for analysis, is poised for a significant transformation with the advent of Generative Artificial Intelligence (Gen AI). Gen AI, a subfield of AI, focuses on creating AI systems that can generate new knowledge and insights. The future implications of Gen AI in data engineering are immense, promising to revolutionize the way we process, analyze, and utilize data.
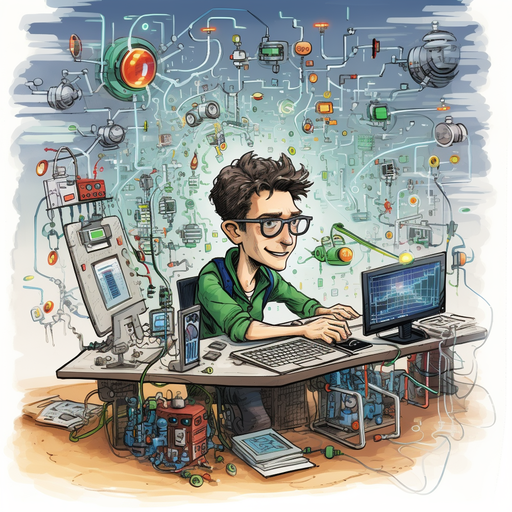
In today’s blog, we will delve deeper into specific aspects of Gen AI's role in data engineering, including enhancing data quality, automating tasks, managing data integration, addressing privacy and security concerns, and the ethical considerations associated with its implementation. By examining these facets, we can gain a comprehensive understanding of how Gen AI is shaping the future of data engineering and its impact on our data-driven world.
The importance of Gen AI:
To understand the importance of Gen AI's future implications in data engineering, let's take a look at some compelling statistics:
- Exponential Growth of Data: According to IBM, around 90% of the world's data has been generated in the past two years alone. This exponential growth in data volume poses challenges for traditional data engineering approaches. Gen AI has the potential to tackle this challenge by automating data processing tasks and extracting valuable insights from vast amounts of data.
- Data Quality Issues: Data quality remains a pressing concern in data engineering. The Data Warehousing Institute estimates that poor data quality costs organizations in the United States around $600 billion annually. Gen AI techniques, such as machine learning algorithms and automated data cleaning processes, can significantly enhance data quality and accuracy, reducing errors and inconsistencies in datasets.
- Need for Automation: Data engineering tasks can be time-consuming and resource-intensive. Gartner predicts that, by 2023, more than 75% of organizations will deploy AI-based automation for data management tasks. Gen AI can automate various data engineering processes, including data integration, transformation, and pipeline creation, freeing up valuable time for data engineers to focus on higher-value tasks.
- Rising Complexity of Data Integration: With the proliferation of data sources and formats, data integration has become increasingly complex. A survey by SnapLogic found that 88% of data professionals face challenges in integrating data from multiple sources. Gen AI can play a vital role in simplifying data integration by leveraging intelligent algorithms to identify data relationships, map schemas, and facilitate seamless integration across diverse datasets.
- Data Privacy and Security Concerns: As data becomes more valuable, ensuring data privacy and security becomes paramount. The World Economic Forum predicts that by 2025, cyber-attacks will result in $10.5 trillion in global damages annually. Gen AI presents both opportunities and challenges in this context. While it can help identify and mitigate potential security risks, it also raises concerns regarding the responsible handling of sensitive data and protecting against algorithmic bias.
Automating Data Engineering Tasks with Gen AI: Benefits and Challenges
Automation is a key driving force behind the advancement of data engineering, and Gen AI offers exciting possibilities for automating various data engineering tasks. By harnessing the power of Gen AI, organizations can streamline their data engineering processes, improve efficiency, and unlock new opportunities. However, along with the benefits, there are also challenges to consider. Let's delve into the benefits and challenges of automating data engineering tasks with Gen AI:
Benefits of Automating Data Engineering Tasks with Gen AI:
- Increased Efficiency: Gen AI can automate repetitive and time-consuming data engineering tasks, such as data extraction, transformation, and loading (ETL), data integration, and data pipeline creation. By automating these tasks, organizations can significantly reduce manual effort, accelerate data processing, and improve overall efficiency in handling large volumes of data.
- Enhanced Accuracy and Consistency: Manual data engineering processes are prone to human errors, which can lead to inconsistencies and inaccuracies in the data. Gen AI techniques, with their ability to process data consistently and precisely, can improve data accuracy, reduce errors, and ensure consistency in data engineering pipelines. This, in turn, contributes to more reliable and trustworthy data analysis.
- Scalability and Adaptability: As data volumes continue to grow exponentially, scalability becomes critical for data engineering. Gen AI-driven automation enables organizations to scale their data engineering processes efficiently. Whether it's handling larger datasets, accommodating new data sources, or adapting to evolving business needs, Gen AI-powered automation provides the flexibility and scalability required to meet these challenges.
- Faster Time-to-Insights: Automation powered by Gen AI accelerates data engineering processes, enabling faster delivery of insights. By reducing manual intervention, organizations can streamline data pipelines, minimize bottlenecks, and expedite the time it takes to transform raw data into actionable insights. This empowers decision-makers with timely and relevant information for making data-driven decisions.
Challenges of Automating Data Engineering Tasks with Gen AI:
- Data Complexity and Variability: Data engineering involves handling diverse data sources, formats, and structures. Gen AI algorithms must be capable of understanding and adapting to this complexity. However, ensuring the accuracy and reliability of automated processes in the face of varied data sources can present challenges. It requires careful validation and testing to account for the nuances of different datasets.
- Data Security and Privacy: Automation brings efficiency but also raises concerns about data security and privacy. With Gen AI automating sensitive data handling tasks, organizations must ensure robust security measures to protect against unauthorized access, data breaches, and potential misuse. Implementing encryption, access controls, and monitoring mechanisms becomes crucial to maintaining data privacy and security.
- Algorithmic Bias and Fairness: Gen AI systems rely on algorithms that learn from historical data. If the training data is biased or reflects existing inequalities, the automated processes can inadvertently perpetuate bias. It is essential to carefully evaluate and mitigate algorithmic bias to ensure fairness and equity in data engineering tasks.
- Skills and Expertise Requirements: Adopting Gen AI for automating data engineering tasks necessitates a skilled workforce. Organizations need data engineers with the expertise to understand and leverage Gen AI technologies effectively. Upskilling and reskilling initiatives are crucial to bridge the skills gap and enable data engineering teams to harness the full potential of Gen AI.
- Legal and Regulatory Compliance: As Gen AI evolves, legal and regulatory frameworks may need to adapt. Organizations must stay updated with evolving regulations related to data privacy, security, and algorithmic transparency. Compliance with these regulations ensures that Gen AI deployment aligns with legal requirements and mitigates potential risks.
By carefully considering these challenges and implementing appropriate strategies, organizations can maximize the advantages of automation while mitigating potential risks.
Exploring the Role of Gen AI in Data Integration and Management
Data integration and management play a crucial role in the success of data engineering initiatives. Gen AI brings forth innovative capabilities that can revolutionize the way organizations handle data integration and management processes. Let's delve into the role of Gen AI in these areas and the benefits it offers:
a. Intelligent Data Integration: Gen AI leverages intelligent algorithms to facilitate seamless data integration from diverse sources. It can automatically identify data relationships, map schemas, and harmonize data formats, allowing organizations to create a unified view of their data. This intelligent integration enables data engineers to access and analyze a comprehensive dataset, unlocking deeper insights and enabling more accurate decision-making.
b. Streamlined Data Transformation: Data transformation involves shaping, cleaning, and structuring raw data to align with specific requirements. Gen AI can automate data transformation processes, reducing manual effort and speeding up the time it takes to prepare data for analysis. With Gen AI, data engineers can define rules and algorithms that automatically transform data, ensuring consistency and quality throughout the transformation process.
c. Enhanced Data Accessibility: Gen AI technologies can improve data accessibility by enabling self-service data access and exploration. With intuitive interfaces and natural language processing capabilities, Gen AI-powered tools allow business users to access and analyze data without relying heavily on data engineers. This empowers organizations to democratize data and foster a data-driven culture across different teams and departments.
d. Real-Time Data Integration: Real-time data integration is becoming increasingly critical today. Gen AI can facilitate real-time data integration by continuously ingesting and processing data as it arrives, ensuring that organizations have access to the most up-to-date information for decision-making. Real-time data integration powered by Gen AI empowers businesses with timely insights and enables them to respond quickly to emerging trends and changing market conditions.
e. Data Governance and Metadata Management: Effective data governance and metadata management are essential for maintaining data quality, compliance, and traceability. Gen AI can assist in automating data governance processes by automatically capturing and documenting metadata, lineage, and data quality metrics. This streamlines data governance and ensures that data remains governed, well-documented, and traceable throughout its lifecycle.
Ensuring Data Privacy and Security in the Era of Gen AI
With the rise of Gen AI in data engineering, ensuring data privacy and security has become more critical than ever. As organizations leverage Gen AI techniques to process and analyze vast amounts of data, it is essential to implement robust measures to safeguard sensitive information. Let's explore the key considerations for ensuring data privacy and security in the era of Gen AI:
a. Secure Data Storage and Transmission: Gen AI relies on data to generate insights, and therefore, it is vital to ensure secure storage and transmission of data. Organizations should employ encryption techniques to protect data at rest and in transit, reducing the risk of unauthorized access or data breaches. Implementing secure protocols and maintaining strong access controls will further enhance data security.
b. Data Minimization and Anonymization: To mitigate privacy risks, organizations should practice data minimization, collecting only the necessary data required for analysis. Gen AI techniques can help anonymize personally identifiable information (PII) by removing direct identifiers or transforming data to ensure individuals cannot be identified. By minimizing data and anonymizing it, organizations can protect individual privacy while still extracting valuable insights.
c. Consent and Ethical Use of Data: With Gen AI processing vast amounts of data, organizations must prioritize obtaining informed consent from individuals whose data is being processed. This includes transparently communicating the purpose and potential outcomes of data analysis. Respecting ethical guidelines and ensuring compliance with data protection regulations becomes paramount to maintain trust and ensure responsible use of data.
d. Robust Access Controls and User Authentication: Controlling access to data is crucial in preventing unauthorized use or manipulation. Organizations should implement robust access controls, ensuring that only authorized personnel can access sensitive data. User authentication mechanisms, such as multi-factor authentication, can add an extra layer of security to prevent unauthorized access to data and Gen AI systems.
e. Algorithmic Bias and Fairness: Gen AI systems learn from historical data, which may contain biases or reflect existing societal inequalities. It is essential to evaluate and mitigate algorithmic bias in data engineering processes. Regular monitoring, rigorous testing, and ensuring diversity and representativeness in training datasets can help address bias and promote fairness in the outcomes generated by Gen AI systems.
f. Regular Auditing and Monitoring: Continuous auditing and monitoring are crucial to detect and address any potential security vulnerabilities or breaches. Organizations should establish monitoring mechanisms to track data access, system activity, and data processing activities. Regular audits of data engineering processes and Gen AI algorithms can help identify and rectify security gaps or compliance issues.
Gen AI Unleashed - Unveiling the Data Engineering Frontier
Gen AI presents immense opportunities to enhance data engineering processes, improve decision-making, and drive business outcomes. However, organizations must navigate the challenges and ethical considerations associated with Gen AI to maximize its benefits responsibly.
As data engineering continues to evolve, embracing Gen AI and addressing its implications will be pivotal in shaping the future of data-driven organizations. By staying informed, adapting to technological advancements, and upholding ethical principles, organizations can unlock the full potential of Gen AI and thrive in the data-driven era.