Kindly fill up the following to try out our sandbox experience. We will get back to you at the earliest.
Data Validation Essential Practices for Accuracy
Discover the importance of data validation in ensuring accuracy and reliability. Learn essential practices and tools for effective data validation.
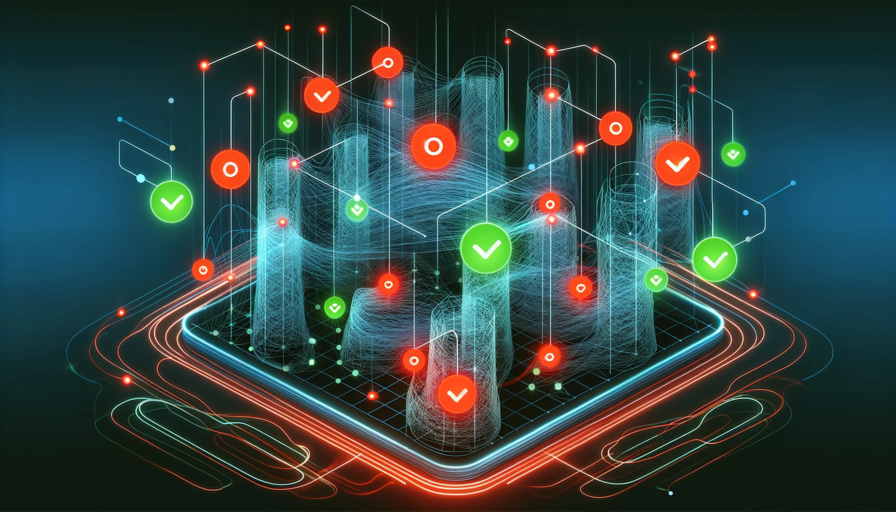
Drive better business insights and decision making with our comprehensive overview of data validation practices, strategies, and the latest tools.
Key Takeaways
- Data validation is essential for ensuring the accuracy and reliability of data.
- Implementing data observability, discovery, and governance tools can facilitate effective data validation processes.
- Utilize a combination of automated and manual data validation techniques to identify and rectify data errors.
- Regularly monitor and audit data validation processes to maintain data integrity.
- Data validation is an ongoing process that requires continuous improvements and updates.
By following these essential practices for data validation, data professionals can ensure that their data is accurate, trustworthy, and reliable, leading to better decision-making and improved business outcomes
Understanding the Importance of Data Validation
Data validation is a linchpin in quality assurance when managing data, assisting in guaranteeing the correctness, completeness, and reliability of data sets. By verifying that data has undergone stringent quality checks, data validation promotes a higher level of data integrity which drives accurate business insights and decision making.
Data validation lifts overall system reliability by detecting errors early, ensuring data integrity, and fueling precise business insights.
In robust database systems, the role of data validation cannot be overstated. It facilitates the detection and correction of errors, significantly reducing the chances of inaccurate data compromising the system. By ensuring data correctness from the onset, data validation curbs the risk of subsequent system malfunctions or inaccuracies in the analysis, enhancing overall system effectiveness and reliability.
The role of data validation in ensuring accuracy
Data validation stands as an essential linchpin in the process of maintaining data integrity. By ensuring the accuracy of data, it optimizes various business operations, paving the way for confident decision-making.
- Promotes high-quality, trustworthy data
- Boosts operational efficiency
- Enhances decision-making confidence
- Supports regulatory compliance
- Minimizes the risk of data-driven errors
The consequences of inaccurate data
The rippling effects of inaccurate data can permeate an organization, often leading to devastating consequences in decision making, operations, customer relations, and compliance.
- Compromised decision-making due to misinformation
- Operational inefficiencies resulting from invalid data
- Degradation of customer trust and satisfaction due to inaccurate insights
- Increased risks of non-compliance and hefty regulatory fines attributed to poor data handling practices
Data Validation Best Practices
Strategizing robust data validation mechanisms requires clear-cut rules that set the bar for data standards. Utilize automated processes to run frequent checks, backed by regular auditing to identify discrepancies and facilitate early intervention.
To execute successful data validation, data professionals need to capitalise on a mix of data profiling techniques and statistical analysis methods. This blend allows them to ensure that the data conforms to established business rules, data types, and defined patterns, thereby ensuring optimal results.
Define clear data validation rules
Defining unambiguous rules for data validation is crucial to avert data corruption. Well-crafted, precise rules enable enhanced data reliability, ensuring the data's versatility and value.
- Incorporation of field-level validation for each data element
- Ensuring consistent data types across the dataset
- Setting minimum and maximum values for numeric fields
- Enforcement of explicit and non-ambiguous data formats
- Application of 'lookup' validations to ensure the data resides in a declared domain
Implement automated data validation processes
Automating data validation processes results in more streamlined and efficient data management, leading to improved data accuracy and reduced human error. This digital transformation introduces a proactive approach to data validation, maintaining the reliability of your datasets while greatly reducing the time and effort required.
- Reduction in human error
- Improved data accuracy
- Efficiency in data management
- Proactive rather than reactive data validation
- Time and cost savings
- Consistent application of data validation rules
Regular data monitoring and auditing
Continuous and systematic data monitoring is crucial in retaining data accuracy. It enables teams to identify unusual patterns and deviations, thereby enhancing data validation.
Data auditing enhances validation by regularly inspecting data sources for inaccuracies and inconsistencies. Through rigorous auditing, organizations maintain reliable data and mitigate risks associated with erroneous information.
Leveraging data profiling techniques
Data profiling techniques hold immense potential to ensure the accuracy of your data assets, acting as a catalyst for validations. These methods not only investigate the general health of your data but shed light on inconsistencies, errors, and missing data instances.
With an enhanced understanding of data distributions and relationships through data profiling, your foundation for robust data validation practices becomes stronger. These insights can be harnessed to detect irregularities and improve the fidelity of your data.
Advanced data profiling techniques fuse quality checks with algorithmic analysis, providing a more in-depth view of your data. Adapting these for your data validation strategy can augment accuracy significantly and improve overall data governance.
Data profiling empowers data professionals with precise insights into the intricacies of data dynamics, from value distribution to perfecting data cleansing techniques. These gleanings resonate across any stages of data preparation, validation, or refinement.
Enhancing the quality of your data requires a dedicated effort beyond just superficial checks. Data profiling ensures the fabric of your data management system is woven tightly, which in turn, bolsters your data validation techniques. The result is higher data accuracy, consistency and reliability.
Using statistical analysis for data validation
Statistical analysis is pivotal in data validation, enhancing precision and accuracy. Through examining trends, correlations, patterns, and anomalies in massive datasets, it provides a robust way to substantiate data accuracy.
Data consistency is verified through statistical methods such as regression analysis or chi-square testing. These methods help to identify discrepancies, enhancing data validity.
Statistical analysis and data validation intersect crucially. Cohesive implementation empowers data professionals to ensure high-quality data outputs, fostering meaningful insights and informed decision-making.
However, the complexity of statistical tools necessitates a sufficient skillset. Therefore, data professionals should prioritize education on statistical methodologies for effective data validation.
Tools and Technologies for Data Validation
Embracing technology, from data observability platforms to machine learning, has revolutionized data validation. These tools improve the accuracy and efficiency of data validation, providing a robust approach to ensuring reliable data outcomes.
To accurately validate data, one must navigate an array of tools and technologies. Utilizing these, from data quality tools to complex data governance platforms, helps in diagnosing inaccuracies, misinterpretations, and ensuring high security standards.
Introduction to data observability platforms
Data observability platforms significantly enhance precise data validation. These platforms provide clear visibility across data pipelines, identifying potential anomalies and facilitating early intervention. Learn what is Data Observability?
Such platforms enable comprehensive data assessment, thereby strengthening validation practices. With in-depth insights into data flows, professionals can refine their validation rules and procedures.
The interplay between data observability platforms and data validation practices fosters effective data quality management. By unifying validation tasks within a single view, these platforms underpin a streamlined, efficient approach to data quality.
This seamless integration guides teams in identifying and solving validation issues promptly on a resource-friendly model. As a result, organizations can rely on accurate, trustworthy data to drive decision-making and maintain competitiveness.
Using data quality tools for data validation
Data quality tools are indispensable in improving data validation efficiency. By promptly identifying and rectifying errors, they save precious time, reduce costly mistakes, and bolster data integrity.
These tools expeditiously untangle the intricate relationship between data validation and quality. They perpetually monitor and refine data, ensuring its compliance with predefined validation rules.
From real-time data checks to automated error reports and amendments, data quality tools add another layer of robustness to your data validation technique. It is this tool-mediated validation that ensures data is not just copious but accurate and useful.
Leveraging machine learning for data validation
Machine learning emerges as a compelling catalyst in streamlining data validation. It unearths complex patterns and errors in data that would otherwise be tedious to identify, significantly enhancing the accuracy and efficiency of verification processes.
Implementing machine learning in data validation equips companies with a predictive edge. It anticipates potential inaccuracies, enabling preemptive measures to correct them, thus heightening the reliability of data-centric decisions.
Moreover, machine learning accelerates data validation, adapting to evolving data dynamics. It learns from each validation cycle, continuously elevating accuracy and diminishing manual dependencies, making data validation strategies more robust and future-proof.
Utilizing data governance platforms
Data governance platforms are pivotal in upholding data validation accuracy. They provide a cohesive framework for defining and implementing data validation rules across various data sources, ensuring uniformity and correctness.
These platforms facilitate accurate data validation by streamlining data-related activities. They establish clear standards for data handling and automate many data validation processes, significantly minimizing human errors.
Platform-induced automation imparts a high degree of consistency and precision to data validation efforts. It ensures that anomalies and inaccuracies get promptly identified, followed by appropriate corrective measures.
With a comprehensive view of the data landscape, these platforms foster a culture of data quality across the entire organization. They make it easier to maintain compliance and meet data-specific regulations.
Data governance platforms also incorporate advanced analytics, making it easier to identify patterns, draw insights and improve validation techniques. Hence, they are an instrumental tool for achieving data validation accuracy.
Addressing Common Challenges in Data Validation
Disparate challenges beleaguer data validation, from missing data to inconsistencies, yet their impact can be mitigated with solid strategies. Crafting reliable procedures for data validation pivots on identifying these often recurrent issues and addressing them with efficiency, while ensuring data security and adeptly managing voluminous data.
Dealing with missing or incomplete data
Strategic measures, such as data imputation and advanced machine learning algorithms, can be deployed to manage missing or incomplete data during validation. These create more robust validation processes and prevent erroneous assumptions in downstream data applications.
Missing or incomplete data compromises the validation process, potentially leading to misconceived insights and substandard decision-making. Methods to mitigate this include regular data auditing and using reliable data discovery tools.
Constant vigilance is critical in handling incomplete data. This includes rigorous observability systems, specific governance processes, and swift corrective measures. As a result, the impact on data quality is minimized and accuracy is preserved.
Handling ambiguous or inconsistent data
When dealing with ambiguous or inconsistent data during validation, one effective strategy is to seek data clarification at the source. This not only resolves ambiguities but also prevents propagation of erroneous information downstream.
Mitigating ambiguity and inconsistency in data validation can be accomplished by implementing a comprehensive data quality management policy. This policy should incorporate standards and rules for every data input, ensuring consistency and reducing risks.
A key challenge is managing ambiguous or inconsistent data within the validation process. Through leveraging tools that offer data observability and discovery, professionals can unravel complexities and promptly rectify inconsistencies.
Ensuring data security and privacy during validation
Securing data privacy during validation is paramount. Trusted practices include user authentication, encryption, pseudonymization, and the principle of least privilege, limiting access to essential personnel only. These measures mitigate unauthorized access and potential data breaches.
Data security plays a critical role in maintaining the integrity of the validation process. By using advanced encryption techniques and network security tools, businesses can ensure the protection of sensitive data.
Protecting privacy during validation often goes hand-in-hand with observance of data handling regulations. These standards, such as GDPR, outline stringent guidelines for data security, ensuring responsible practice during validation processes.
Implementing privacy by design is an effective practice. This strategy integrates data protection principles from the onset of system design, building security directly into the validation process.
Validation doesn't have to compromise security. Access controls, audit trails, and regular security reviews can ensure the protection and privacy of the data being validated, whilst maintaining integrity and accuracy.
Managing large volumes of data during validation
Navigating the complexities of managing large data volumes during validation can be arduous. In order to ensure the seamlessness of this task, powerful and proficient data tools become indispensable. This alleviates the burden of processing colossal data sets and ensures validation accuracy.
An excellent strategy for managing large data volumes during validation is to divide the data into manageable portions. This 'divide and conquer' approach allows for more focused and efficient validation, and reduces the risk of overwhelming the system.
Another potent strategy is the utilization of parallel processing. By distributing tasks and running them concurrently, data validation can be scaled up, speeding the process while maintaining the quality of results.
Cloud-computing platforms, known for their scalability and cost-effectiveness, provide an excellent solution for validating large data volumes. They can accommodate growing data needs while providing sturdy security measures, vital for the protection of enterprise data.
Conclusion
Data validation is a crucial aspect of data management and analysis. By implementing the essential practices outlined in this article, data professionals can ensure the accuracy, reliability, and integrity of their data. Data observability, discovery, and governance tools play a significant role in facilitating effective data validation processes.
Automated and manual data validation techniques should be employed to identify and rectify data errors. Regular monitoring and auditing of data validation processes are necessary to maintain data integrity.
Data validation is not a one-time task but an ongoing process that requires continuous improvements and updates. By following these best practices, data professionals can have confidence in the quality of their data, leading to better decision-making and improved business outcomes.
Remember, data accuracy is paramount in today's data-driven world, and data validation is the foundation for achieving that accuracy.