Kindly fill up the following to try out our sandbox experience. We will get back to you at the earliest.
What is Data trust? Maturity Framework
Discover steps to achieve reliable data across the value chain, focusing on trust beyond tools—essential for data products and GenAI.
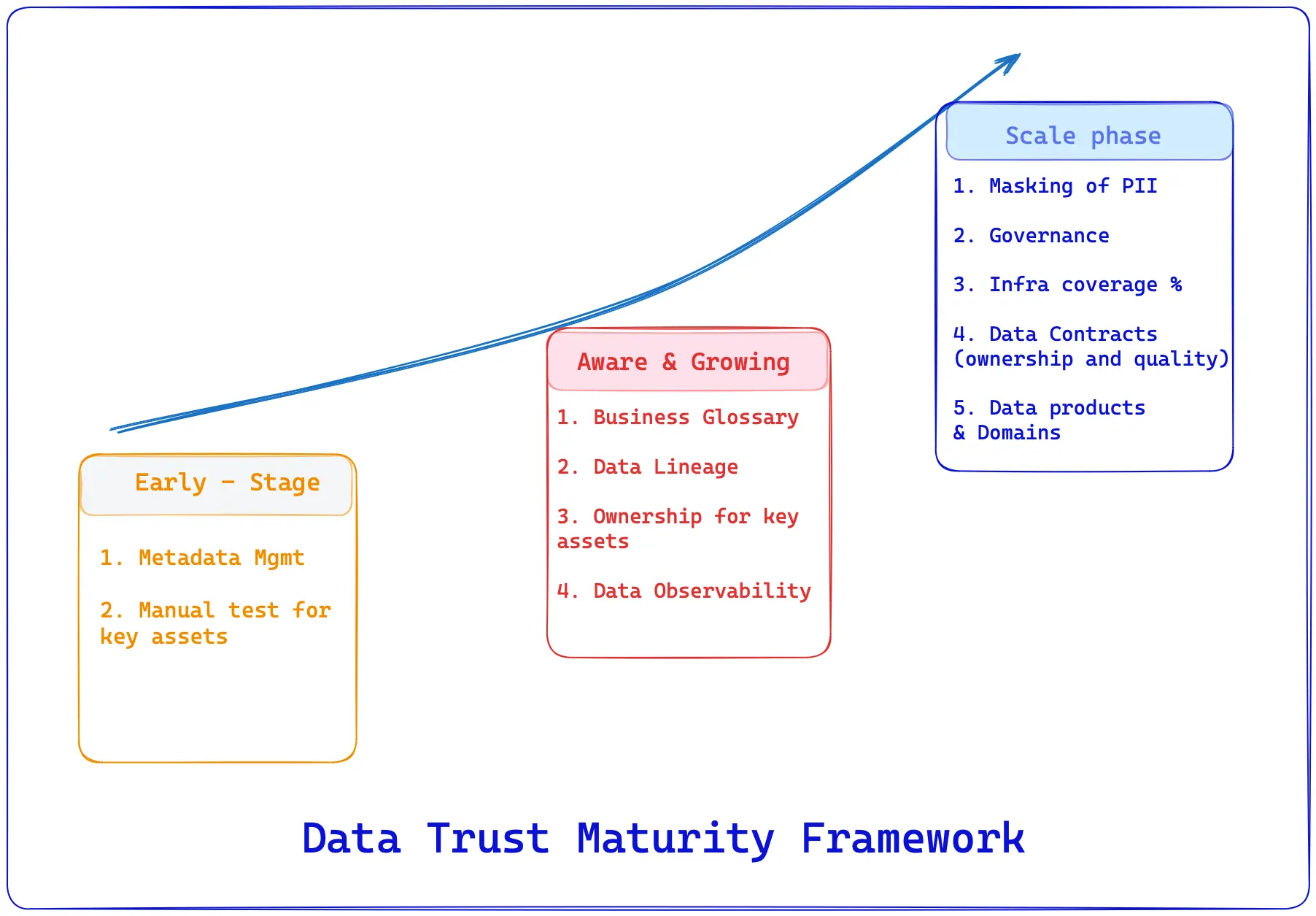
In the ever-evolving landscape of data management, the concept of Data Trust has emerged as a cornerstone for organizations seeking to harness the full potential of their data assets. As data engineers, we are well aware that trust in data is not a static state but a dynamic condition that grows and matures over time. To guide organizations through this journey, we present the Data Trust Maturity Framework, a roadmap designed to evolve from the early stages of data management to a sophisticated, scalable data governance ecosystem.
Key Takeaways
- Data Trust is Dynamic: Trust in data evolves and matures with an organization, and it is critical to establish a framework to guide this evolution.
- Foundation is Key: Early-stage practices like metadata management and manual testing of key assets are essential to understand and document the data environment.
- Growth Through Visibility: The Aware & Growing phase is characterized by establishing a common data language, tracking data lineage, and defining data ownership—all leading to proactive data management.
- Scaling Up: The Scale phase involves advanced practices like PII masking, data governance, and the creation of data products, indicating a mature data trust environment.
- Culture Over Tools: Implementing the Data Trust Maturity Framework is as much about cultural change as it is about tools and processes, requiring time and dedication.
- Strategic Differentiator: A strong Data Trust framework transforms data into a strategic differentiator, enabling innovation and informed decision-making.
Early Stage: Laying the Foundations
At the outset of a data journey, organizations often find themselves managing metadata and conducting manual tests for key assets. While this phase is characterized by a more reactive approach to data issues, it is crucial for establishing the groundwork for more advanced data trust practices.
Metadata Management
Metadata management is the process of handling data about data. At this stage, it is crucial to begin cataloging data sources, defining data models, and understanding the relationships between different data entities. This foundational step ensures that there is a basic level of understanding and documentation that can be built upon in the future.
Manual Test for Key Assets
Manual testing involves the rigorous examination of key data assets to ensure their accuracy, consistency, and reliability. Although labor-intensive, this process is vital for early detection of issues and serves as a hands-on approach to understanding the data landscape.
Aware & Growing: Building Trust
As organizations mature, they move into the Aware & Growing phase, where the focus shifts to a more proactive approach to data management. This phase is marked by the introduction of a business glossary, data lineage, assignment of data ownership, and the beginnings of data observability.
Business Glossary
A business glossary is a curated list of terms and definitions that are critical to an organization's data landscape. It serves as a shared language, ensuring that all stakeholders have a common understanding of data-related terms, which is essential for effective communication and collaboration.
Data Lineage
Understanding data lineage is akin to tracing the life story of data. It involves mapping out the journey of data from its origin through various transformations to its final form. This visibility is key to maintaining data quality and diagnosing issues quickly.
Ownership for Key Assets
Assigning ownership to key data assets ensures accountability and fosters a sense of responsibility for maintaining data quality. Data owners are tasked with the stewardship of their data, setting policies, and enforcing standards that contribute to the overall trust in the data.
Data Observability
Data observability marks the beginning of a systematic approach to monitoring data systems. It encompasses tracking the health of data pipelines, staying alert to anomalies, and gaining insights into the performance of the data ecosystem.
Scale Phase: Advanced Data Trust Practices
In the Scale phase, the organization has established a comprehensive data trust system that is both robust and adaptable. This phase introduces advanced practices such as masking personally identifiable information (PII), formal governance structures, infrastructure coverage, data contracts, and the creation of data products and domains.
Masking of PII
As data privacy becomes increasingly paramount, the ability to mask PII is a critical component of the Scale phase. It involves implementing techniques to protect sensitive information while still allowing for the utility of the data.
Governance
Data governance is the formal orchestration of people, processes, and technology to enable an organization to leverage data as an enterprise asset. It includes establishing clear policies, standards, and procedures that govern the use of data within the organization.
Infra Coverage %
This metric gauges the extent to which the data infrastructure is monitored and governed. A high percentage indicates a mature data trust environment where the infrastructure is thoroughly understood and controlled.
Data Contracts (Ownership and Quality)
Data contracts are formal agreements that outline the expectations for data quality and the responsibilities of data owners. They serve as a binding framework that ensures all parties are aligned on the standards to which the data must adhere.
Data Products & Domains
At this advanced stage, data is treated as a product, with deliberate design and maintenance aimed at meeting the needs of its users. Data domains are established to organize data into logical groups, allowing for more effective management and discovery.
Conclusion
The Data Trust Maturity Framework presents a structured path for data engineers to evolve their data practices. By understanding and implementing this framework, organizations can move from a rudimentary stage of data management to a sophisticated, trust-based data ecosystem. This evolution not only enhances the reliability of data but also empowers organizations to make informed decisions, innovate, and maintain a competitive edge in today's data-driven world.
For data engineers, the journey through the Data Trust Maturity Framework is not just about implementing tools and processes; it is about fostering a culture that values data quality, transparency, and accountability. As we embrace this journey, we lay the foundation for an environment where data is not just an asset but a trusted ally in achieving organizational goals.