Kindly fill up the following to try out our sandbox experience. We will get back to you at the earliest.
Data Observability: Maximize LLM Accuracy
Explore how Data Observability enhances LLM accuracy by improving data quality, model performance, and empowering data scientists. Revolutionize machine learning
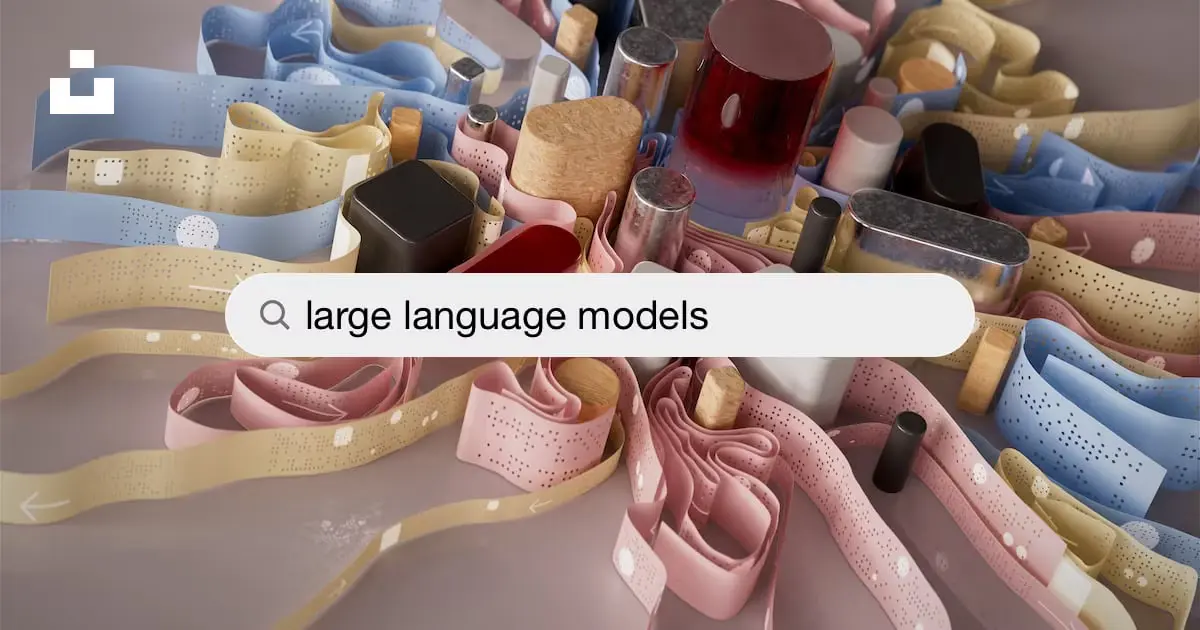
Introduction to data observability
As the field of artificial intelligence continues to advance, so does the need for accurate and reliable language and learning models (LLM). These models are designed to process and understand human language, enabling machines to perform tasks such as chatbots, language translation, and text summarization. However, ensuring the accuracy of LLM models poses several challenges. That's where data observability comes into play.
Understanding LLM models
Before diving into data observability, it's important to understand what LLM models are and how they work. LLM models are a type of machine learning model that utilize large language models to process and analyze textual data. These models are trained on massive amounts of data to learn patterns, structures, and meanings in language. By doing so, they can generate human-like responses and make predictions.
The importance of accuracy in LLM models
Accuracy is crucial when it comes to LLM models. Whether it's a chatbot providing customer support or an automated language translation system, the accuracy of the model directly impacts its performance and usefulness. If an LLM model consistently generates incorrect or nonsensical responses, it can lead to frustrated users and a loss of trust in the system. Therefore, maximizing the accuracy of LLM models is paramount.
Challenges in maintaining accuracy in LLM models
Maintaining accuracy in LLM models can be challenging due to various reasons. Firstly, language is complex and ever-evolving. New words, slang, and cultural references emerge constantly, making it difficult for models to keep up. In addition, biases present in the training data can be learned and perpetuated by the model, leading to biased or unfair responses. Furthermore, the context in which language is used can greatly affect its meaning, and models may struggle to understand and interpret context accurately.
What is data observability?
Data observability is the practice of monitoring, understanding, and ensuring the quality, reliability, and accuracy of data used in machine learning models. It involves tracking and analyzing data throughout its lifecycle, from collection to preprocessing and model training. By implementing data observability, organizations can identify and rectify issues that may impact the accuracy of LLM models.
How data observability maximizes the accuracy of LLM models
Data observability plays a vital role in maximizing the accuracy of LLM models. Firstly, it enables organizations to identify and address biases in the training data. By monitoring the data and the model's responses, any biases can be detected and mitigated, ensuring fair and unbiased outcomes. Additionally, data observability allows organizations to track and measure the performance of their LLM models over time. By analyzing metrics such as accuracy, precision, and recall, organizations can pinpoint areas for improvement and fine-tune the models accordingly.
Techniques and tools for implementing data observability
Implementing data observability requires the use of various techniques and tools. Firstly, organizations need to establish comprehensive data tracking and logging mechanisms. This involves recording and storing information about the data used for training, including its source, quality, and any preprocessing steps applied. Organizations can also utilize data validation techniques, such as anomaly detection and data profiling, to identify and rectify issues in the data. Furthermore, tools like data quality monitoring dashboards and automated data validation pipelines can provide real-time insights into the health and accuracy of the data.
Case studies showcasing the impact of data observability on LLM models
Several case studies demonstrate the impact of data observability on the accuracy of LLM models. For example, a healthcare organization used data observability techniques to identify biases in their language translation model. By addressing these biases, they were able to provide more accurate translations, improving patient care and safety. Another case study involved a customer support chatbot that was continuously generating incorrect responses. Through data observability, the organization discovered a flaw in the data collection process, leading to better training data and improved accuracy in customer interactions.
Future trends in data observability for LLM models
As the field of data observability continues to evolve, several future trends are expected to emerge. Firstly, there will be an increased focus on interpretability and explainability of LLM models. Organizations will seek ways to understand how these models arrive at their decisions and predictions, ensuring transparency and accountability. Additionally, advancements in natural language processing techniques will enable more accurate and context-aware LLM models. Finally, the integration of automated data validation and monitoring tools into the model training pipeline will streamline the process of ensuring data accuracy and reliability.
Conclusion
Data observability is a game-changer when it comes to maximizing the accuracy of LLM models. By monitoring and ensuring the quality, reliability, and accuracy of the data used in these models, organizations can address biases, track performance, and ultimately provide more accurate and reliable language and learning solutions. As the field of artificial intelligence continues to advance, data observability will play a crucial role in building trustworthy and effective LLM models.