Kindly fill up the following to try out our sandbox experience. We will get back to you at the earliest.
Data Lineage : Examples, Concepts and Techniques
Explore the essentials of Data Lineage to understand its impact on data governance, management, and how it enhances trust in your data ecosystems.
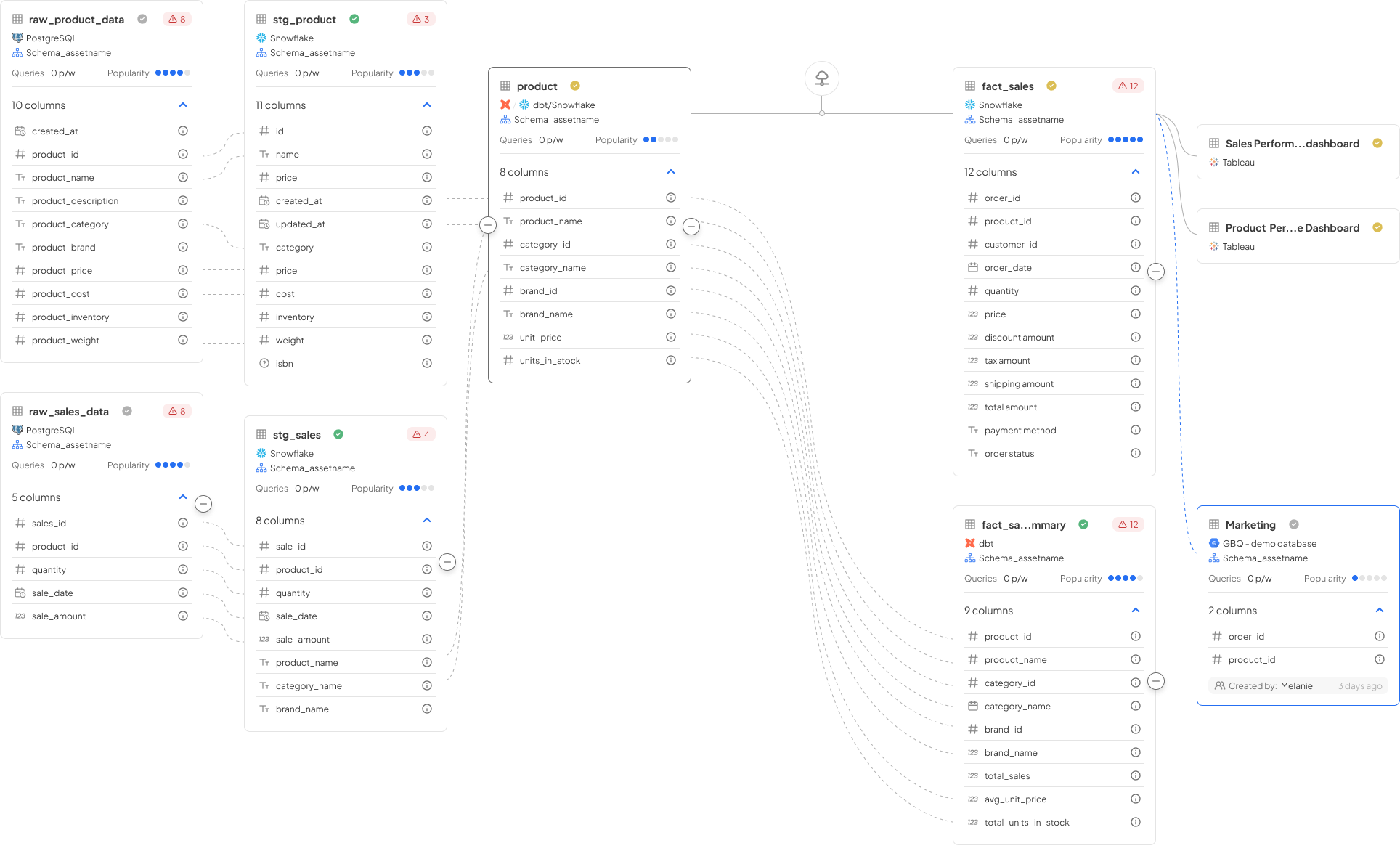
Data Lineage - Concepts Explained
So, what exactly is data lineage? Data lineage refers to the process of tracking and documenting the origins and transformations of data throughout its lifecycle. It allows organizations to understand where data comes from, how it is processed and manipulated, and how it flows across various systems and applications. By establishing a robust data lineage framework, businesses can gain valuable insights into their data, make informed decisions, and maintain regulatory compliance.
Data lineage plays a vital role in data governance and management by enhancing data quality, supporting compliance efforts, and facilitating effective decision-making processes. It provides a clear and transparent view of data lineage ensures the accuracy and reliability of data, which is critical for organizations operating in today's data-driven landscape.
In this article, we will explore the key components and processes involved in establishing data lineage, discuss the importance of data lineage in data governance, and highlight best practices for implementing and managing data lineage effectively. We will also delve into the tools and software available for data lineage management, the data lineage process, and its application in big data environments and data observability.
Don Tapscott, the author of "Blockchain Revolution," puts it: "Data is the new oil. It's valuable, but if unrefined, it cannot really be used." Data lineage is one of the most effective ways to refine and optimize your organization's data management processes.
Key Takeaways:
- Data lineage is the process of tracking and documenting the origins and transformations of data.
- Data lineage enhances data quality, supports compliance efforts, and enables effective decision-making.
- Implementing data lineage requires a robust framework, best practices, and specialized tools and software.
- Data lineage is applicable in big data environments and plays a crucial role in data observability.
- Data lineage strengthens data governance practices, ensuring compliance and effective data management.
Understanding Data Lineage
When it comes to data management, understanding the concept of data lineage is crucial. Data lineage refers to the tracking of data from its origins to its transformations throughout its entire lifecycle. By following the journey of data, organizations can gain valuable insights into data quality, data governance, and decision-making processes.
Data lineage provides a comprehensive view of how data moves and changes within an organization's systems. It helps answer questions such as:
"Where did this data come from?"
"How was this data transformed?"
"What processes and systems have accessed or modified this data?"
By answering these questions, data lineage offers transparency and traceability, which are essential for ensuring data accuracy, compliance, and trustworthiness.
The Key Components of Data Lineage
A robust data lineage framework consists of several key components that work together to provide a complete understanding of data origins, transformations, and usage. These components include:
- Data Sources: The systems or applications that generate or serve as the origin of the data.
- Data Transformations: The processes or operations that modify or enrich the data as it moves through various stages.
- Data Destinations: The systems or applications that consume or utilize the data.
- Metadata: Information about the data, including its structure, format, and context.
These components form the building blocks of a data lineage framework, enabling organizations to map and understand the flow of data throughout their data ecosystems.
The Process of Establishing Data Lineage
Establishing a robust data lineage framework involves several essential processes, including:
- Data Discovery: Identifying and cataloging the data sources within an organization's systems.
- Data Mapping: Documenting the relationships and dependencies between data sources, transformations, and destinations.
- Data Documentation: Creating detailed documentation that captures metadata and other relevant information about the data.
- Data Validation: Verifying the accuracy and integrity of the data lineage framework through audits and verification processes.
By following these processes, organizations can establish a solid foundation for understanding and managing data lineage effectively.
Benefits of Understanding Data Lineage
- Enhanced Data Quality
- Improved Compliance
- Support for Decision-Making Processes
Data lineage offers numerous benefits to organizations. By understanding the origins and transformations of data, organizations can:
- Ensure data accuracy and reliability, leading to enhanced data quality.
- Meet compliance requirements by tracing data lineage and demonstrating data governance practices.
- Make informed decisions based on a thorough understanding of data sources and transformations.
Overall, understanding data lineage is vital for organizations seeking to build trust, ensure data integrity, and optimize their data management efforts.
The Importance of Data Lineage
When it comes to data governance and management, data lineage plays a crucial role in ensuring data accuracy, trustworthiness, and compliance. By tracking the origins and transformations of data throughout its lifecycle, data lineage provides organizations with a clear understanding of how data is created, manipulated, and used.
Data lineage enhances data quality by enabling organizations to identify data anomalies, inconsistencies, and errors. It allows for better data validation, ensuring that only reliable and accurate data is used for decision-making processes.
Effective compliance is another benefit of data lineage. With data lineage, organizations can trace the origin of data, facilitating audits, regulatory reporting, and adherence to data privacy and security regulations. This transparency and traceability help organizations demonstrate compliance and mitigate potential risks.
Data lineage also supports the decision-making process. By providing insights into the data's lineage, organizations can evaluate the reliability and validity of the data, reducing decision-making risks. Additionally, data lineage enables organizations to identify relationships between different data sets, facilitating data-driven analyses and strategic planning.
Data lineage is of paramount importance in data governance and management, bringing numerous benefits to organizations. By enhancing data quality, enabling compliance, and supporting decision-making processes, data lineage empowers organizations to leverage their data effectively and confidently.
Data Lineage Benefits:
- Enhances data quality and accuracy
- Enables effective compliance and risk mitigation
- Supports decision-making processes
- Fosters data-driven analyses and strategic planning
Data Lineage Best Practices
In order to effectively implement and manage data lineage, it is important to follow a set of best practices. These practices will enable you to capture and maintain data lineage, ensure data accuracy, and address common challenges that arise in data lineage initiatives. Here are some key best practices to consider:
- Start with a clear understanding of your data: Before establishing data lineage, it is essential to have a thorough understanding of your data assets. Conduct a comprehensive data inventory and analysis to identify all relevant data sources, transformations, and dependencies. This foundational knowledge will serve as the basis for establishing and maintaining accurate data lineage.
- Establish standardized documentation processes: Consistent and standardized documentation is crucial for effective data lineage management. Develop clear guidelines and templates for documenting data lineage, including naming conventions, data source descriptions, and transformation rules. This will ensure that everyone involved in the data lineage process understands and follows the documentation requirements.
- Implement data lineage capture mechanisms: Invest in tools and technologies that can capture and trace data lineage throughout its lifecycle. These mechanisms should automatically track data movement and transformations, providing a real-time view of data lineage. Consider using metadata management platforms or specialized data lineage software to facilitate this process.
- Regularly validate and verify data lineage: Data lineage is only valuable if it is accurate and up to date. Implement procedures for regularly validating and verifying the captured data lineage. This can include conducting data lineage audits, cross-referencing with source systems, and performing data lineage integrity checks. Identify and resolve any discrepancies or errors to maintain the reliability and integrity of your data lineage.
- Collaborate with data owners and stakeholders: Establish a collaborative relationship with data owners and key stakeholders throughout the organization. Engage in regular communication to understand their data needs, challenges, and expectations. By involving them in the data lineage process, you can ensure that the captured lineage aligns with their requirements and addresses any specific data governance concerns.
By following these best practices, you can optimize your data lineage initiatives and derive maximum value from your data assets. Implementing effective data lineage practices will enhance data accuracy, promote trustworthiness, and enable better decision-making within your organization.
Data Lineage Tools:
The Features and Functionalities of Data Lineage Tools
Data lineage tools offer a wide range of features and functionalities that enable organizations to effectively manage their data lineage initiatives. Some key capabilities of these tools include:
- Automated data discovery: Data lineage tools can automatically discover and capture data sources, ensuring comprehensive coverage of the data ecosystem.
- Data mapping and visualization: These tools provide graphical representations of data lineage, allowing users to easily understand the flow of data across systems and processes.
- Metadata management: Data lineage tools offer robust metadata management capabilities, enabling organizations to capture important information about data assets.
- Impact analysis: With data lineage tools, organizations can perform impact analysis to understand how changes in data sources or transformations impact downstream systems and processes.
- Version control and change management: These tools facilitate version control and change management for data lineage documentation, ensuring accuracy and accountability.
The Benefits of Using Data Lineage Software
By utilizing data lineage software, organizations can unlock a host of benefits that contribute to their overall data governance and management efforts. Some key advantages include:
- Improved data quality: Data lineage software helps organizations identify and rectify data quality issues by tracing data back to its source and identifying any transformations or changes along the way.
- Enhanced compliance: With data lineage software, organizations can demonstrate data lineage to regulators and auditors, ensuring compliance with data regulations and standards.
- Efficient decision-making: By providing clear visibility into the origins of data, data lineage software empowers organizations to make informed decisions based on reliable and trustworthy data.
- Streamlined data governance: Data lineage software plays a key role in establishing a robust data governance framework, enabling organizations to effectively manage data-related risks and enforce data policies.
- Auditing and traceability: With data lineage software, organizations can easily audit data flows and track changes, facilitating traceability and accountability.
Data Lineage Process
In order to establish a robust data lineage management system, organizations need to follow a well-defined data lineage process. This process involves several key steps that ensure the accurate tracking and documentation of data origins and transformations. By implementing these steps, organizations can enhance their data governance practices and maintain data accuracy and trustworthiness.
Data Discovery
The data discovery phase is the initial step in the data lineage process. It involves identifying and cataloging all relevant data sources within an organization. During this phase, data engineers and analysts work together to locate and document the various systems, databases, and files that house data. This step is critical for understanding the overall data landscape and capturing the initial data lineage.
Data Mapping
Once the data sources have been identified, the next step is to map the flow of data between these sources. Data mapping involves tracing the movement of data from its origin to its destination, including any transformations or modifications along the way. This step helps create a visual representation of the data lineage, showing how data moves through different stages of processing.
Data Documentation
Documenting the data lineage is essential for maintaining transparency and ensuring data accuracy. During this phase, organizations capture and record the details of the data lineage, including source systems, data transformations, and destinations. This documentation serves as a valuable reference for data governance, compliance, and decision-making processes.
Data Validation
The final step in the data lineage process is data validation. This involves verifying the accuracy and completeness of the captured data lineage. Data validation techniques may include comparing the documented lineage with actual data movements, conducting data quality checks, and performing reconciliation processes. By validating the data lineage, organizations can ensure its reliability and make informed decisions based on trustworthy data.
Implementing a comprehensive data lineage process is crucial for effective data lineage management. By following these steps, organizations can establish a reliable data lineage framework that enhances data governance, supports compliance efforts, and enables better decision-making.
Data Lineage in Big Data Environments
In the world of big data, where vast amounts of data are generated and processed at unprecedented speed, data lineage plays a crucial role in ensuring data accuracy, reliability, and trustworthiness. Managing data lineage in big data environments presents unique challenges and considerations that organizations must address to maintain data integrity.
One of the key challenges in managing data lineage in big data environments is the handling of distributed data. With data spread across multiple nodes and systems, tracking the flow and transformations of data becomes more complex. Organizations need sophisticated techniques and tools to capture and trace lineage across distributed data sources and platforms.
Another challenge is dealing with diverse data sources. Big data ecosystems often involve various types of data, including structured, semi-structured, and unstructured data from different sources such as databases, cloud storage, social media, IoT devices, and external sources. Establishing a data lineage across these diverse sources requires comprehensive data discovery and mapping techniques.
Despite these challenges, effectively managing data lineage in big data environments offers significant benefits. It enables organizations to gain a holistic view of their data landscape, ensuring data quality, compliance with regulations, and facilitating data-driven decision-making processes. By understanding the lineage of data, organizations can identify potential bottlenecks, anomalies, and data quality issues, allowing them to take proactive measures to rectify any issues.
"In big data environments, data lineage serves as a navigational guide, illuminating the path data takes, helping organizations gain insights, ensure data quality, and maintain trust in their data ecosystems."
To overcome the challenges and realize these benefits, organizations can adopt various techniques to manage data lineage in big data environments:
- Implementing automated data lineage processes that leverage machine learning and artificial intelligence (AI) algorithms to identify and track the flow of data.
- Utilizing metadata management solutions to capture and store comprehensive metadata about data sources, transformations, and lineage relationships.
- Applying data governance practices and policies to ensure data lineage aligns with organizational data management strategies and compliance requirements.
- Employing data cataloging tools that integrate with data lineage solutions to provide a unified view of data assets, their lineage, and related metadata.
By employing these techniques, organizations can establish a robust data lineage framework in big data environments, enabling them to effectively manage data quality, compliance, and decision-making processes, while gaining a comprehensive understanding of their data assets.
Challenges and Techniques in Managing Data Lineage in Big Data Environments
Data Lineage in Data Observability
Data observability is a critical component of modern data management practices. It focuses on monitoring and understanding data behaviors and anomalies to ensure data reliability and quality. In this section, we will explore the role of data lineage in data observability and discuss how it helps organizations maintain the trust and accuracy of their data.
Data lineage, as we have discussed earlier in this article, is the process of tracking and documenting the origins and transformations of data throughout its lifecycle. It provides organizations with a clear understanding of how data moves and changes, enabling them to identify potential issues and ensure data integrity.
When it comes to data observability, data lineage plays a crucial role in several ways:
- Ensuring Data Reliability: By tracing the lineage of data, organizations can verify the source and validity of their data. This helps in identifying any discrepancies or inaccuracies in the data, ensuring that the data being observed is reliable and trustworthy.
- Identifying Data Drift: Data lineage allows organizations to detect any unexpected changes or deviations in the data flow. By comparing the current state of the data with its lineage, data drift can be identified and investigated promptly, minimizing the impact on data observability.
- Maintaining Data Quality: Data lineage helps in maintaining the quality of the observed data. By understanding the data's journey and transformations, organizations can implement proper data quality controls and proactively address any issues that may impact data observability.
"Data lineage provides a granular view of data movements and transformations, empowering organizations to ensure data reliability, identify data drift, and maintain data quality in their data observability practices."
By incorporating data lineage into their data observability frameworks, organizations can establish a robust foundation for monitoring, analyzing, and improving the health and performance of their data ecosystem. It enables them to identify and resolve data-related issues quickly, ensuring that the observed data remains accurate, consistent, and reliable.
Data lineage empowers organizations to achieve comprehensive data observability, enabling them to make informed decisions, identify potential risks, and ensure the accuracy and reliability of their data. In the next section, we will explore specific data lineage techniques that data engineers can employ to establish effective data lineage frameworks.
Data Lineage and Data Catalog
In the realm of data management, data lineage and data catalog are two interconnected concepts that play a crucial role in understanding and governing data assets. Data lineage provides a comprehensive trail that traces the origins, transformations, and relationships of data, while data catalog serves as a centralized repository for metadata and information about datasets.
When combined, data lineage and data catalog create a powerful synergy that enables organizations to gain deep insights into their data assets. Data lineage enhances data cataloging efforts by providing valuable context and history about the data, empowering data stewards and analysts to make informed decisions.
Benefits of Data Lineage in Data Cataloging:
- Enhances data understanding: Data lineage enriches the metadata available in the data catalog by illustrating the flow of data across various systems, applications, and processes. This detailed information fosters a better understanding of the data, helping users interpret its meaning and establish data trustworthiness.
- Improves data quality and accuracy: By visualizing the transformations and interactions that occur throughout the data lifecycle, data lineage uncovers potential sources of errors or inconsistencies. This enables organizations to identify and rectify data quality issues, ensuring that the data catalog contains reliable and accurate information.
- Streamlines data governance: Data lineage supports effective data governance practices, enabling organizations to establish control mechanisms, enforce compliance requirements, and manage data-related risks. By incorporating data lineage into the data catalog, organizations can strengthen their data governance frameworks and ensure adherence to regulatory standards.
The integration of data lineage and data catalog is facilitated by metadata management. Metadata serves as the foundation for both concepts, providing essential information about datasets, such as data source, schema, transformations applied, and data usage policies. Effective metadata management ensures that data lineage and data catalog initiatives are successful, promoting a holistic understanding of data assets.
By harnessing the power of data lineage and data catalog, organizations can unlock the full potential of their data assets. These practices foster data-driven decision-making, enhance data trustworthiness, and promote efficient data governance. As the data landscape continues to evolve, integrating data lineage and data catalog will become increasingly critical for organizations seeking to gain a competitive edge through data-driven insights.
Rounding Up
Data lineage plays a crucial role in data governance and management. Throughout this article, we have emphasized the significance of data lineage in enhancing trust and confidence in data ecosystems. By tracking the origins and transformations of data, organizations can ensure data accuracy, improve decision-making processes, and achieve regulatory compliance.
Furthermore, data lineage works hand in hand with data catalog tools and effective data management practices. Data catalog tools provide a centralized repository for organizing and documenting data assets, enabling easy discovery and understanding of data lineage. When combined with robust data management practices, such as data quality control, data lineage becomes even more valuable in ensuring data integrity and reliability.
To successfully implement data lineage, organizations should consider leveraging data catalog tools that support data lineage capabilities. These tools help streamline the capture, maintenance, and visualization of data lineage, making it easier for businesses to manage their data assets effectively. Additionally, employing proper data management practices enhances the overall data governance framework, ensuring the accuracy, security, and compliance of data.
FAQ
What is data lineage?
Data lineage refers to the process of tracking and documenting the origins, transformations, and destinations of data throughout its lifecycle. It provides a comprehensive view of how data flows within systems, ensuring data accuracy, integrity, and trustworthiness.
Why is data lineage important?
Data lineage is important because it enhances data governance and management practices. It helps organizations understand the data they possess, how it is transformed, and how it is used. Data lineage ensures data quality, supports decision-making processes, and facilitates compliance with regulations and policies.
What are the benefits of data lineage?
Data lineage offers several benefits to organizations, including improved data quality, enhanced data governance, increased trust and confidence in data, better compliance with regulations, improved decision-making processes, and efficient data troubleshooting and debugging.
What are some best practices for implementing data lineage?
Some best practices for implementing data lineage include capturing and maintaining data lineage information in a centralized repository, involving stakeholders from various departments, documenting data transformations and business rules, regularly validating data lineage accuracy, and aligning data lineage efforts with overall data governance strategies.
What tools and software are available for data lineage management?
There are several tools and software solutions available for data lineage management, such as Collibra, Informatica Axon Data Governance, Alation, MANTA, and lineage visualization tools like erwin Data Modeler and IBM InfoSphere Information Governance Catalog. These tools assist in capturing, documenting, visualizing, and managing data lineage information.
What is the data lineage process?
The data lineage process involves several steps, including data discovery, data mapping, data documentation, data validation, and data visualization. These steps help in understanding the origin, transformation, and usage of data, ensuring data accuracy and integrity.
How does data lineage apply to big data environments?
In big data environments, data lineage becomes more complex due to the volume, velocity, and variety of data. Data lineage in big data focuses on capturing the lineage of data across distributed systems and diverse data sources. Techniques such as metadata management, data tagging, and automated data lineage tools are employed to manage data lineage in these environments.
What role does data lineage play in data observability?
Data lineage plays a crucial role in data observability by helping organizations monitor and understand data behaviors and anomalies. It enables organizations to identify data quality issues, detect data drift, and ensure reliable and trustworthy data for analysis and decision-making.
What data lineage techniques are useful for data engineers?
Data engineers can employ various techniques for effective data lineage management, including using data lineage integration frameworks, automating data lineage capture and maintenance processes, implementing scalable data lineage solutions, and leveraging metadata management practices. These techniques help data engineers design and maintain robust data lineage frameworks.
How does data lineage relate to data catalog?
Data lineage and data catalog are closely related. Data lineage enhances data cataloging efforts by providing information on the origin, transformations, and destinations of data, thus establishing the lineage of data assets. Metadata management is key to both data lineage and data catalog initiatives, as it helps in capturing and organizing metadata associated with data assets.
If you need help implementing data lineage, talk to us today!