Kindly fill up the following to try out our sandbox experience. We will get back to you at the earliest.
Data Quality Guide: Accurate, Consistent, Reliable Data Management
Explore the importance of data quality in organizations and how effective data management systems can mitigate risks from inaccurate data.
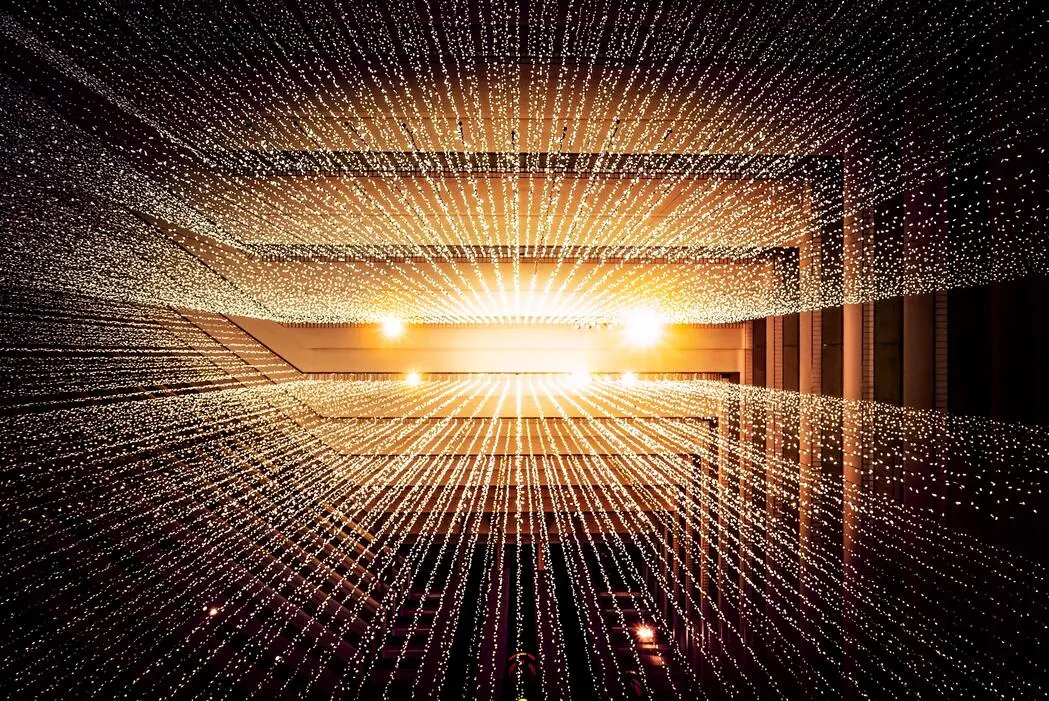
Data Quality Guide
Data, needless to say, is the lifeline of any organization, be it an e-commerce or a fintech organization. It's the driving force behind crucial business decisions and the foundation upon which organizations build their success. Unfortunately, many organizations often ignore the significance of data quality and the consequences of poor data management, even though it has such an important role.
Recent studies have shown that poor data quality costs organizations billions annually. According to Statista, global IT spending on data center systems is anticipated to reach 222 billion USD in 2023. But poor data quality can result in up to 60% of business decisions being based on inaccurate data. A study about Data Quality found that organizations lose an average of 12% of their revenue due to poor data quality.
Aren't these numbers scary? Despite these alarming statistics, many organizations still struggle with data quality management. Organizations generate vast data but lack standardization and manage data from multiple sources, making data quality management challenging and complex. So how do we overcome this?
Implementing accurate data quality management systems can help the organization overcome all these challenges. In this blog, let us delve into how to design and build that perfect Data Quality Management system for your organization.
Building a Data Quality Management System: That actually works;
The cornerstone of a successful data quality management system is a well-defined framework that includes clear policies, procedures, and metrics. The success lies not only in implementing this framework but by regularly reviewing and updating it to stay relevant and effective. Additionally, fintech companies should establish data quality metrics and KPIs to measure the success of their data quality efforts. So all of this contributes to building a data quality Management system.
Though building a Data Quality Management (DQM) system is a critical step for organizations, it is a systematic approach to ensure that data is accurate, complete, and consistent and meets the organization's requirements. To achieve this, you may consider asking the below and take the appropriate steps:
- Data Quality Requirements: The first step in building a DQM system is to define the data quality requirements of the organization. This includes identifying what data type is important to the organization, what quality standards are expected, and what measures will be used to determine data quality.
- Is Data Governance in place? Data governance is a critical component of any DQM system, as it provides the framework for managing and monitoring data quality. This includes establishing policies and procedures for data management, defining roles and responsibilities for data management, and ensuring that data quality is a top priority for all stakeholders.
- Implement Data Quality Controls: Once the data quality requirements and governance structure are in place, organizations can implement data quality controls to ensure that data meets quality standards. This may include implementing data validation rules, establishing data entry procedures, and implementing data cleaning and enrichment processes to improve data accuracy and completeness.
- Monitor Data Quality: It is a critical step in maintaining a DQM system. This includes regular monitoring of data quality metrics, such as data accuracy, completeness, and consistency, and taking corrective actions as needed to improve data quality.
- Move towards continuous improvement:Building a DQM system is not a one-time event but an ongoing process. Organizations should continuously evaluate their data quality and make improvements as necessary to ensure that their data meets their evolving needs and requirements.
Though the above establishes the essential groundwork for your data quality management systems, it may need to include a few more necessary steps. Let us cover them below.
We are talking about data catalogs and observability. These two emerging technologies have the potential to drastically improve the way organizations manage and make use of their data. Let us understand what these are and how they can help.
- Data Catalog: A centralized repository of information about an organization's data assets provides an overview of the data sources, data definitions, and relationships between data elements. Organizations can quickly discover and understand the data gaps by having a complete picture of all data assets, leading to improved decision-making and reduced data waste.
- Observability: Observability enables organizations to understand how data flows through their systems, identify potential issues, and take corrective actions to improve data quality. By implementing observability practices, organizations can gain greater insight into the performance of their data management systems, allowing them to make informed decisions about data quality and improve the overall data management process.
By combining data catalogs and observability, over and above the basic data quality management system, organizations can build a comprehensive data management system that is both efficient and effective. So basically a foolproof system.
Decube, a data management company focused on providing cutting-edge solutions to organizations looking to improve their data management processes, provides integration of data catalogs and observability into its product. Now that is a game changer for organizations looking to improve their data management processes. The integration of data catalogs and observability into a single solution is what sets Decube apart from other companies in the industry. This combination provides organizations with a comprehensive and efficient data management system unmatched by any other company.
After building a data quality management system, it is crucial to have other parts ready. What are those? Let us cover them here;
Other essential processes of a Data Quality management system are:
1. Data Cleaning and Enrichment Techniques: Data cleaning and enrichment are two critical processes in ensuring data quality. Data cleaning, as the name suggests, involves removing errors, inconsistencies, and inaccuracies from data, while data enrichment involves adding missing information or enhancing existing data. Both go hand in hand to make sense of the data.
2. Training and Education for Data Quality Management:To maintain high data quality standards, it is important to educate employees on data quality best practices. This education should be ongoing and include regular training sessions, resources, and support.
Additionally, organizations should establish a data quality culture where employees understand the importance of data quality and take ownership of their role in maintaining it. This is as important as the DQM itself. Remember that well-trained employees can bring the change you are looking for!
The Future of Data Quality: Embrace the change
Stay ahead of the curve in the ever-evolving world of data quality. With new technologies and regulations emerging, it's crucial to keep up to date on the latest trends. Artificial intelligence and machine learning are revolutionizing how we manage data quality, while regulations like open banking and PSD2 are transforming how we handle data. Not only that but embracing change and transforming is the key to the future of data quality.
Data Quality is the key to Successful Data Management:
By implementing a comprehensive data quality management system, regularly training employees, and staying abreast of the latest developments in data quality, fintech companies can ensure that their data is accurate, consistent, and reliable. This, in turn, will enable them to make informed decisions, improve the customer experience, and ultimately drive business success.
If you're looking for a comprehensive and effective solution to improve your data management processes, ask your tech partners if they have a data catalog and observability in their offerings. In fact, with the integration of data catalogs and observability, Decube is the only company that can provide you with the complete data management solution you need to succeed.
Learn more about Decube here