Kindly fill up the following to try out our sandbox experience. We will get back to you at the earliest.
Data Observability Use Cases
Explore the transformative power of data observability use cases - from data quality monitoring to anomaly detection and lineage tracking for effective data management.

Data Observability Use case
In less than three years, data observability has rapidly moved up the technology ladder. It now sits at the top, marking its quick success among the latest tech trends. We explored numerous data observability projects to find 61 uses and benefits that real companies enjoy. These uses, while varied in popularity, have universally improved how businesses handle their data.
Key Takeaways
- Data observability has seen rapid growth, moving from an idea to reaching the Gartner Hype Cycle in less than three years.
- A review of hundreds of data observability deployments identified 61 real-world use cases and benefits.
- Data observability has helped organizations like Resident, Contentsquare, BlaBlaCar, and Choozle reduce data incidents, detection times, and overall data downtime.
- Data observability platforms can detect issues that traditional alerting systems miss, such as silent data delivery failures and schema changes.
- Data observability is a growing element of data infrastructure, with 83.9% of executives planning to increase investment in data and analytics this year.
Introduction to Data Observability
Data observability is crucial for an organization to understand its data's health and quality. It helps make their data ecosystem strong. This is done by letting data teams keep an eye on the quality, reliability, and how data is delivered. They can also spot and solve problems.
By making data observability a priority, organizations gain many benefits. These include better data quality, less data downtime, and quick problem fixes. Yet, setting up data observability isn't always easy. Challenges come from too much data, confusing signals, and teams who don't work together.
What is Data Observability?
Data observability lets us keep track of how healthy our data is, inside our organization's systems. It allows data teams to watch over how data is sent, if it's dependable, and its quality. This way, any issues that pop up can be noticed and solved fast.
Benefits of Data Observability
Data observability offers big pluses by making data's condition visible. It brings about better data quality, less data downtime, and quicker problem resolutions. For instance, Choozle saw an 80% drop in data downtime, thanks to this approach. In another case, Contentsquare cut the time to notice data issues by 17% in just one month.
Challenges in Implementing Data Observability
The upsides of data observability are great, but so are the hurdles. Organizations must tackle issues like more data, complicated tools, and teams that don't share info. These problems can make getting a clear view of data health tough. It's vital that enterprises address these challenges. Only then can they fully use the power of data observability for better data management and data governance.
Data Observability Use Cases
One key use case of data observability is to make data better. It does this by cutting down the time when data isn't right. This might happen when data is wrong, missing, or not available. Systems for data observability use smart tools to spot problems early. They tell data teams everything they need to know. This has cut the time to find an issue by 17% in just one month for a company like Contentsquare. Other companies, such as Resident, BlaBlaCar, and Choozle, have seen fewer issues with their data thanks to this method.
Improving Data Quality
A study with 200 data pros found it takes longer to fix data problems each year. It used to be 9 hours but now it's 15. By using data observability, BlaBlaCar got much faster at solving these problems. Their speed improved by 50%. Choozle also saw a big change. They had 80% less time with bad data thanks to this technology.
Reducing Data Downtime
Checkout.com, a fintech company, keeps an eye on over 4,600 data sets. They help over 300 users a day with their data systems. Plus, they work on 1,200 dbt models regularly. This shows how big data observability's impact can be. These systems can find problems that are hard for people to catch. For example, spotting empty queries affecting many data sets was a big help. Data observability also lets users watch out for strange data. They can find issues that stop decisions being made quickly, like missing customer info.
Identifying and Resolving Data Issues
An event where three tables got changed but gained no new data was flagged right away by data observability. This shows its power to find small but important data issues. Looking closely at specific customer details helped find strange values. For example, finding zero instead of expected numbers. This led to fixing the main cause of these data quality problems. Data observability is vital for spotting problems in modern data tools. It helps keep data in good shape all along its journey. For example, tools like Fivetran, dbt, and Airflow. This keeps data accurate as it moves through different systems. Mercari’s story is a great example. They used data observability to quickly deal with mistakes in their data flow. This shows how it's key to keeping data fresh and correct in complex systems.
Detecting Unexpected Schema Changes
Data observability helps with spotting sudden changes in data's structure. This is key because such changes might break the flow of data further down the line. The problem is data engineers often can't control where the data comes from. This can lead to big troubles with how data is used.
Mercari and Freshly, big online shops, quickly noticed when their data schemas broke. Thanks to tools like data observability, they fixed the problems fast. With these tools, engineers could also predict how changes might affect their end users.
Monitoring Schema Changes in Production Databases
These tools can alert you to problems early. They can detect changes in data quality fast, which can lower costs and stop issues before they spread. For instance, a drop in data accuracy by 60% was caught, showing the tools' power. By using these tools, you can watch out for all sorts of issues. They keep an eye out for weird data or if a data set's size changes unexpectedly. This quick notice lets you investigate issues right away.
Planning for Schema Changes in Data Warehouses
In a big data system, knowing where problems start is crucial. Data lineage helps you follow these issues back to the root cause. This is vital for making sure your data is reliable.
Setting up these monitoring tools is simple. They check data quality all the time and alert you as needed. This prevents problems from spreading to other parts of the system.
Ensuring Data Freshness
Today, every company uses data for decisions. So, keeping data fresh is super important. With the help of data observability, checking on data often is easier. This is very helpful for big companies with lots of data. For example, Checkout.com watches over 4,600 data sets. They support more than 300 users. And they use over 1,200 models, all to keep their data fresh.
Automating Data Freshness Monitoring
Setting up data freshness alerts isn't too hard. But, making sure they work for a big company is tough. That's where data observability steps in. It makes checking data's freshness across a whole company simple. This helps teams find and fix problems early. This could be issues like sudden changes in data or how it's organized. These issues might hurt data's freshness and its quality for later use.
Scaling Data Freshness Alerts
Companies are dealing with more and more data. So, keeping an eye on data's freshness is key. This is where solutions for data observability come in. They let organizations look after data freshness over the entire data library. This stops old data from messing up decision-making. But, making all this work is a big deal of work too.
Monitoring Data Volume Anomalies
Think of tables like Goldilocks–the number of rows must be just right to avoid red flags. Too few or too many can be trouble. By using data volume monitors in data observability, issues like empty queries become easier to spot. For instance, a company updated three tables with no new rows. The platform caught this, warning about downstream impact swiftly.
Spotting unusual amounts of data is key for observability tools. They watch table sizes closely to catch any odd gaps. This helps many companies lower their data errors and downtime across time, like Resident, BlaBlaCar, and Choozle.
If data amounts are off, it might mean a problem in the data flow. It could be a schema change or another hidden issue. Data observability gives data teams the power to quickly find and fix these issues. This stops them from causing more problems further down the line.
Data tools do more than just find problems. They guess how many queries could be hit by a single data issue. For example, one issue affected 200 queries. This points out how crucial it is to monitor and solve problems quickly.
Tracking Field-Level Data Anomalies
Tracking your data goes beyond just checking its general health. It lets you focus on specific areas with issues that might hurt data quality. If data doesn't fit its usual patterns or if NULL values suddenly change, there's probably a quality problem to fix.
Monitoring Null Rates
Data observability lets you keep an eye on null values at a detailed level. For example, finding zeroes in fields like "device type ID" or "patron ID" can show problems. Since null values can mess with unique ID associations, spotting these issues early helps keep data clean. This proactive approach lets teams nip data quality issues in the bud before they spread.
Monitoring Unique Value Rates
Observability also means watching how many unique values appear in certain fields. Big shifts in these counts might flag a data quality issue to look into. By focusing on individual fields, data teams can solve these anomalies quicker. This detailed approach ensures the integrity of critical business data remains intact.
Observing System-Level Issues
Many parts of the modern data stack can send alerts when a problem occurs. But, these alerts often miss some key points. They're not always able to spot if a task succeeded but used bad data. Data observability platforms step in here. They find system-wide issues that usual alerts overlook. Mercari's platform, for instance, spotted a failure in a streaming pipeline. This mistake affected lots of tables. Thanks to this find, the team fixed the problem fast. These platforms are also great at finding silent data delivery failures. For instance, they can spot when a Salesforce password runs out. This can make a data table stop updating, but it might not be noticed. Data observability makes sure these issues don't sneak past.
Monitoring Data Pipeline Failures
Data observability platforms let teams see how well their data pipelines are performing. They highlight issues fast, so data flow is not interrupted. They do this by watching important stats and sniffing out weird patterns. This way, teams can flag problems early. These can be things like issues with pipeline connections or bottlenecks in the infrastructure. Catching these problems early means data users won't be affected later.
Detecting Silent Data Delivery Failures
Data observability is also great at catching quieter data issues. For example, it can notice when a Salesforce password expires. This can make a data table go stale. Traditional tools might miss this. Data observability understands data flow deeply. This helps it find these hidden issues before they cause bigger problems.
Data Observability Use Cases
Data observability is key for many things. It boosts data quality, management, and how data is kept safe. It's great for making your data flow better, making sure data is good, and dealing with changes over time. This helps in many areas, including making things run smoother and fixing problems easier. It lets us track where data comes from, spot issues, and always get better. It also helps to make sure folks trust the data, follow rules, and work more effectively.
There are ten big areas data tools should cover. They include getting ready, running things, making changes, and managing costs. Experts say we should focus on what's good for both the money we spend and the benefits we get. This is how we win with data observability. This method keeps an eye on how accurate and fast your data is and if things are working as they should.
But, there are problems too. Things like too much data, misleading signals, and everyone working alone. Companies can do a lot better by improving how they work with data. This makes them more efficient and their work more reliable. It means business projects keep going and are less likely to have problems with deadlines. This makes their work more valuable and lets them move quicker in changing times.
Let's break down what data observability does. It helps set up your data system, fine-tune how it runs, and make sure it keeps up. Then, it looks at how to best store data, making things move when needed, and keeping an eye on the costs. By planning ahead, we can make sure we have enough resources, use them well, and know how to grow. And, it helps make our data routes more efficient so things get where they should go in the best way.
Identifying Code-Level Issues
Data downtime and the types of data issues are big factors to consider. It's key to understand why these problems happen in the code. Platforms for observing data can find query issues and changes in queries. These often start data issues. So, data teams can fix problems fast, saving others from being affected.
Detecting Query Failures
Platforms for watching data can see if there's a query that doesn't work. When queries don’t work, they can mess up the data we see. Keeping an eye on how well queries do lets data teams find and fix issues. Doing this helps make sure the data we use is reliable for important business choices.
Tracking Query Changes
Watching for query failures is important. And so is keeping track of how queries change over time. Even small changes to queries can cause big problems in the data. Things like missing data or wrong numbers can pop up. By keeping an eye on changes, data teams can catch problems early. Then, they can decide when to update or change their data systems.
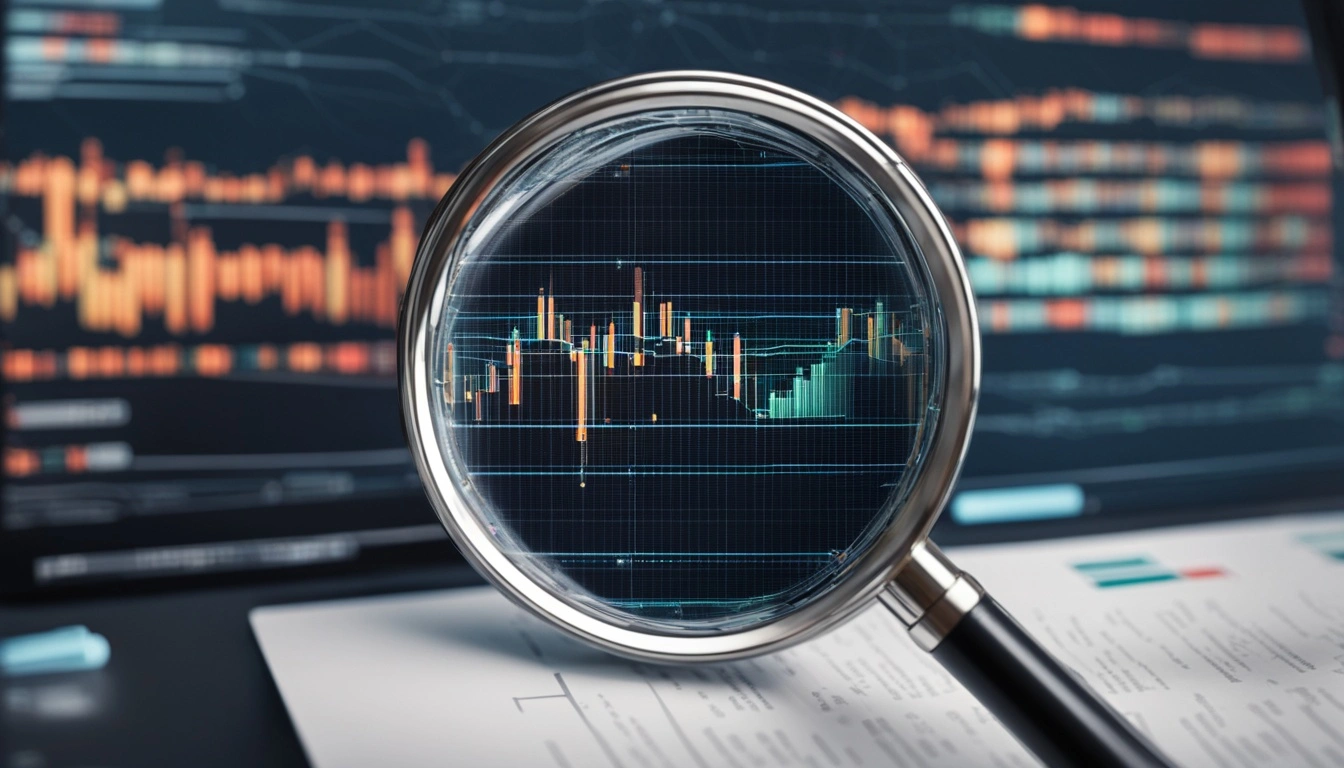
Using tools to look deep into code helps catch the start of data issues. Finding and dealing with these early keeps data reliable. This means better decisions can be made using the data. It lets a company really make the most of their data.
Analyzing Data-Level Anomalies
Data observability helps spot data-level anomalies by checking how data values change over time. It’s key for keeping an eye on changes in null rates or unique values. These changes might point to data quality issues. For example, wide spikes in null rates could signal a problem. Plus, data drift, which means small changes in training data's characteristics over time, can be seen and managed early on with the right tools. This keeps important business applications running with accurate data.
Monitoring Value Distributions
It’s crucial to monitor how data values are distributed. This lets companies catch hidden data quality problems. By checking for sudden changes in a dataset’s values, we can find problems such as spiking null rates or shifting unique values. This early spotting helps fix issues quickly. It also ensures that data used for making business choices and analytics is reliable.
Detecting Data Drift
Data drift can harm the performance of machine learning models or other data apps over time. Observability tools are crucial for spotting and handling data drift. By watching for shifts in data, we can tell when algorithm training data no longer matches the live data. This triggers the need for a model update. Doing this often maintains the precision of data-dependent business tools.
Conclusion
Data observability is a key tool for data teams to make their data better. It helps them see data quality, how data is sent, and the performance of pipelines. With this tool, companies can find and fix problems, check if data is fresh and accurate, and trust their data. Using data and analytics well is important for businesses. So, having a strong data observability plan is key. This plan can help make better data-based decisions and get the most value from data.
Data observability tools have many features. They can deal with data quality issues like sudden changes in structure, keep an eye on data freshness, and spot odd data. These tools show when there are problems at different levels. This lets data teams act fast to keep data reliable and trusted.
As the need for data-driven decisions keeps increasing, a good data observability program is vital. It gives the necessary view and understanding to keep data high-quality and dependable. This can help both data teams and those making business decisions to be better informed. Plus, it can lead to long-lasting success for the business.
FAQ
What is data observability?
Data observability means understanding the health and quality of data in systems. It lets data teams check data quality and find issues. This makes the data ecosystem strong.
What are the benefits of data observability?
It improves data quality, prevents data downtime, and speeds up fixing issues. With it, data teams and businesses can make smarter choices using good data.
What are the common data observability use cases?
Use cases vary, from making data pipelines stable and fast to ensuring data quality. It covers tuning for performance and tracking data lineage. It aims to build trust in data, meet rules, and improve efficiency.
How can data observability help with unexpected schema changes?
Observability technology spots schema changes and lets teams know fast. This helps fix problems before affecting data users. It also shows how changes will affect data projects.
How does data observability ensure data freshness?
It checks data freshness across an organization, ensuring timely, useful data. This stops old data from affecting decisions.
How can data observability detect data volume anomalies?
It uses monitors to find data volume issues like missing info or big changes in row numbers. Finding these early helps fix data quality problems.
What field-level data anomalies can data observability track?
It looks for unusual changes at the field level, like big jumps in null rates. This can point to data quality problems that need fixing.
How can data observability observe system-level issues?
This system spots big issues like broken streaming pipes that regular checks might miss. It helps solve problems fast and keeps data users happy.
How does data observability help identify code-level issues?
It finds errors in queries and tracks query changes, which often cause data issues. This lets teams quickly fix code-level problems.
How can data observability analyze data-level anomalies?
Its tools track data changes and ensure the data behind big business apps stays correct. This way, important data stays trustworthy over time.